SEATTLE, Jan. 27, 2022 — Researchers at the University of Washington (UW) have developed optical computing hardware for AI and machine learning that is faster and more energy efficient than conventional electronics-based computers. The work also addresses another challenge: the “noise” that is inherent to optical computing and that can interfere with computational precision.
The team demonstrated that its system can mitigate noise and that it can use some of it as an input to help enhance the creative output of the artificial neural network within the system.
“We’ve built an optical computer that is faster than a conventional digital computer,” said lead author Changming Wu, a UW doctoral student in electrical and computer engineering. “And also, this optical computer can create new things based on random inputs generated from the optical noise that most researchers tried to evade.”
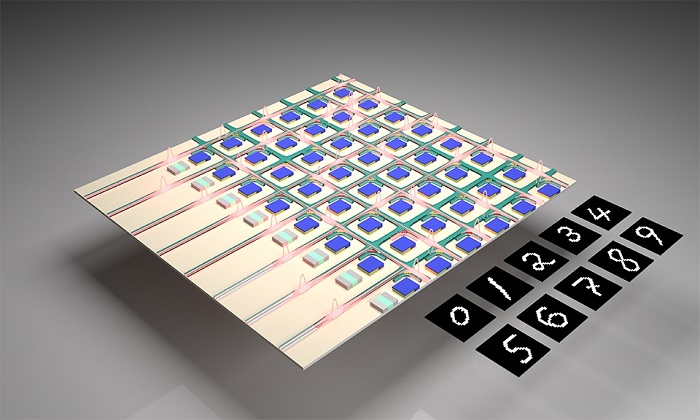
An illustration of the UW-led research team’s integrated optical computing chip and “handwritten” numbers it generated. The chip contains an artificial neural network that can learn how to write like a human in its own, distinct style. This optical computing system also uses “noise” (stray photons from lasers and thermal background radiation) to augment its creative capabilities. Courtesy of Changming Wu, University of Washington.
Optical computing noise comes from stray photons that originate from the operation of lasers within the device and background thermal radiation. To target the noise, the team connected its optical computing core to a special type of machine learning network called a generative adversarial network (GAN).
The team tested several noise mitigation techniques, which included some of the noise generated by the optical computing core to serve as random inputs for the GAN. For example, the team assigned the GAN the task of learning how to handwrite the number “7” like a person would. The optical computer could not simply print out the number according to a prescribed font; it had to learn the task in a way similar to how a child would, by looking at samples of handwriting and practicing until it could write the number correctly. The computer did this by generating digital images with a style similar to the samples it had studied but not identical.
“Instead of training the network to read handwritten numbers, we trained the network to learn to write numbers, mimicking visual samples of handwriting that it was trained on,” said senior author Mo Li, a UW professor of electrical and computer engineering. “We, with the help of our computer science collaborators at Duke University, also showed that the GAN can mitigate the negative impact of the optical computing hardware noises by using a training algorithm that is robust to errors and noises. More than that, the network actually uses the noises as random input that is needed to generate output instances.”
The next steps include building the device at a larger scale using current semiconductor manufacturing technology. The team plans to use an industrial semiconductor foundry to achieve wafer-scale technology, rather than construct the next iteration of the device in a lab. A larger-scale device will improve performance and allow the research team to do more complex tasks beyond handwriting generation such as creating artwork and even videos.
“This optical system represents a computer hardware architecture that can enhance the creativity of artificial neural networks used in AI and machine learning, but more importantly, it demonstrates the viability for this system at a large scale where noise and errors can be mitigated and even harnessed,” Li said. “AI applications are growing so fast that in the future, their energy consumption will be unsustainable. This technology has the potential to help reduce that energy consumption, making AI and machine learning environmentally sustainable — and very fast, achieving higher performance overall.”
The research was published in Science Advances (www.doi.org/10.1126/sciadv.abm2956).