Neural Field Network Reconstructs High-Resolution from Low-Resolution Images
Deep learning (DL) has significantly transformed the field of computational imaging, offering solutions to enhance performance and address a variety of challenges. Traditional methods often rely on discrete pixel representations, which limit resolution and fail to capture the continuous and multiscale nature of physical objects.
To address this problem, researchers from Boston University (BU)’s Computational Imaging Systems Lab have introduced a local conditional neural field (LCNF) network. Called neural phase retrieval (NeuPh), the LCNF network leverages advanced DL techniques to reconstruct high-resolution phase information from low-resolution measurements.
This method employs a convolutional neural network (CNN)-based encoder to compress captured images into a compact latent-space representation. Then, this is followed by a multilayer perceptron (MLP)-based decoder that reconstructs high-resolution phase values, effectively capturing multiscale object information. By doing so, NeuPh provides resolution enhancement and outperforms both traditional physical model-based methods and current state-of-the-art neural networks.
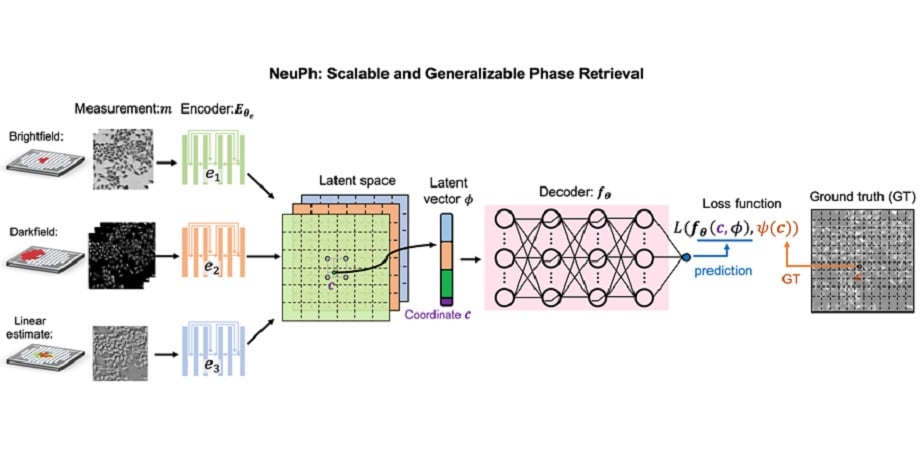
A diagram showing NeuPh’s scalable and generalizable phase retrieval. The LCNF network uses a CNN-based encoder to learn and encode measurement information into a latent-space representation, while a MLP decoder reconstructs the phase values at specific locations with an increased spatial resolution by synthesizing local conditional information from the corresponding latent vectors. Courtesy of H. Wang et al., doi 10.1117/1.APN.3.5.056005.
The reported results highlight NeuPh’s ability to apply continuous and smooth priors to the reconstruction, showcasing more accurate results compared to existing models. Using experimental datasets, the researchers demonstrated that NeuPh can accurately reconstruct intricate subcellular structures; eliminate common artifacts such as residual phase unwrapping errors, noise, and background artifacts; and maintain high accuracy even with limited or imperfect training data.
NeuPh also exhibits strong generalization capabilities. It consistently performs high-resolution reconstructions when trained with very limited data or under different experimental conditions. This adaptability is further enhanced by training on physics-model-simulated datasets, which allows NeuPh to generalize well to real experimental data. According to lead researcher Hao Wang, the researchers tried to combine experimental and simulated datasets as a hybrid training strategy to ensure effective network training.
“NeuPh facilitates ‘super-resolution’ reconstruction, surpassing the diffraction limit of input measurements,” Wang said. “By utilizing ‘super-resolved’ latent information during training, NeuPh achieves scalable and generalizable high-resolution image reconstruction from low-resolution intensity images, applicable to a wide range of objects with varying spatial scales and resolutions.”
The research was published in Advanced Photonics Nexus (www.doi.org/10.1117/1.APN.3.5.056005).
Published: September 2024