Early diagnosis of musculoskeletal diseases is vital for effective treatment. Near-IR spectroscopy (NIRS) is emerging as a technique for more accurate quantitative assessment.
JAAKKO SARIN, UNIVERSITY OF EASTERN FINLAND
As the aging population continues to grow, the number of people suffering from musculoskeletal diseases, such as osteoarthritis (OA), is expected to drastically increase. The symptoms of OA are diverse and include pain, soreness, and stiffness of affected joints. These symptoms, however, may only begin to manifest in the later stages of the disease, at which point the most common treatment involves partial or total joint replacement.

Arthroscopic NIRS measurements in the equine joint in vivo. The NIRS probe is aligned perpendicular to articular cartilage by monitoring with an arthroscope (camera). NIRS-probe alignment on cartilage surface (inset). Courtesy of Biophysics of Bone and Cartilage/University of Eastern Finland.
In idiopathic OA, the underlying cause is often unknown, although risk and prevalence of the disease have been associated with numerous factors, such as age, genetics, gender, muscle weakness, obesity, dietary intake, and bone density. OA can also be triggered by physical trauma to the joint. This is typically referred to as post-traumatic OA (PTOA). Unlike idiopathic OA, development and progression of PTOA can be halted if the disease is detected early.
To prevent disease progression,
endoscopy-guided surgeries are performed to repair torn ligaments, the meniscus, and damaged cartilage. The current diagnostic tools available to orthopedic surgeons during arthroscopic procedures are limited to visual evaluation and manual probing of the tissue (above). Such approaches are subjective and unreliable, as highlighted by an opinion survey among highly experienced arthroscopic surgeons1, and cannot provide information beyond the tissue surface.
A potential solution for objectively assessing joint tissue conditions during arthroscopy is the use of optical spectroscopy, such as near-IR spectroscopy (NIRS). Despite the early introduction of NIRS in the 1940s, the nonspecificity of overlapping spectral features in the near-IR spectral region limited its application. Nowadays, multivariate modeling and deep neural networks can be used to extract important spectral features in the visible and near-IR regions for predicting target properties from the spectral data.
For example, current state-of-the-art image classification networks, such as automatic driving-aid software, use convolutional neural networks to detect learned features (such as pedestrians) in images and video. Similarly, convolutional neural networks have recently shown great promise in the analysis of one-dimensional spectral data, with potential for the estimation of tissue-specific parameters from near-IR spectra.
Tissue diagnostics
NIRS is a nondestructive optical-sensing technique that can be applied in various measurement geometries, such as reflectance and through-transmission modes. In medical applications, the reflectance mode is more commonly used.
In certain geometrically optimal anatomical sites, however, such as fingers or earlobes, through-transmission can be used. As the penetration depth of light depends on its wavelength2, this limits and guides the choice of optimal measurement geometry. The near-IR region covers the wavelengths from 700 to 2500 nm (12,500 to 4000 cm−1), which includes first, second, and third overtone bond vibrations (bending and stretching) of organic compounds.
The relative intensity of these vibrations in the near-IR is weaker compared to the mid-IR region. However, the mid-IR region is only capable of penetrating up to a few micrometers into soft tissues, making diagnosis of deep tissue layers impossible. With NIRS, more thorough diagnosis is possible because of the better penetration depth (up to 5 mm) of light into soft tissues.
The NIRS measurement system can be employed for diagnosis of any tissue where changes in tissue structure and composition are biomarkers of disease.
A reflectance NIRS system generally consists of three hardware components: spectrometer, light source, and fiber optic probe. Prior to tissue measurements, background light and hardware-related noise are accounted for by measuring the spectrum of a nonreflective material. In addition, to ensure the highest possible signal-to-noise ratio, acquisition times of spectrometers are optimized while measuring the response from a reflectance standard, typically one that reflects over 99% of light.
In medical applications, the main technical issue is related to a low signal-to-noise ratio (SNR), usually because of the short acquisition times necessary for real-time operation. Although the SNR can be improved by increasing the light-source intensity or sensitivity of the detector (mainly the noise of read-out electronics), optimizing the propagation of light into and out of the target tissue is most important. The optimization of light propagation is primarily governed by the design of the optical probe. Its form and function depend on the type of optical fibers used in its construction.
In an attempt to develop an optimal and ergonomic probe for arthroscopic procedures, a novel sterilizable probe, which resembles the shape of a conventional arthroscopic hook used by orthopedic surgeons worldwide, was developed at the University of Eastern Finland (UEF) (Figure 1). The system was designed for repeated use, with no interchangeable or single-use parts, ensuring a relatively low cost of usage. The near-IR detectors, such as indium gallium arsenide (InGaAs) and germanium (Ge) photodiode detectors are the most expensive hardware components of the measurement system.
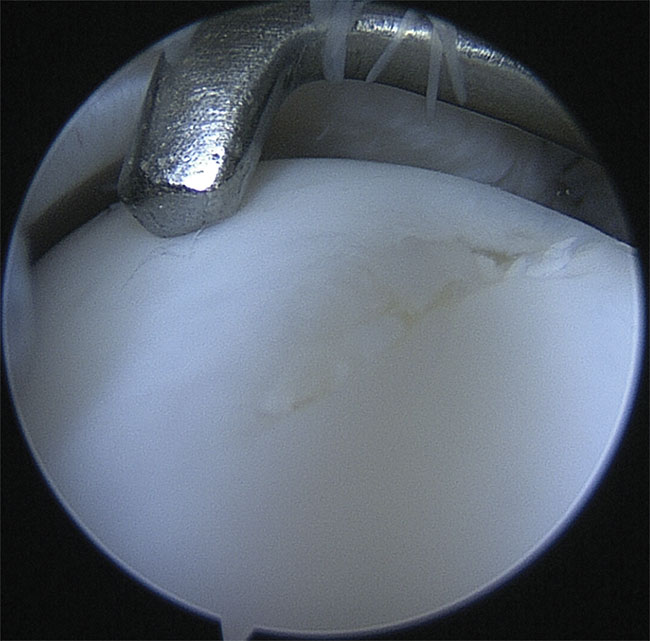
Figure 1. Visual evaluation of articular cartilage with a conventional arthroscope (camera), along with the assessment of the tissue’s functional integrity by palpation with a metallic hook. Courtesy of the Department of Equine Sciences/Utrecht University.
Applications in medicine
Although established clinical use of NIRS is scarce, the technique has great potential for various medical applications, such as screening for skin cancer. The Biophysics of Bone and Cartilage research group of UEF has focused on using the technique for improving the diagnosis of joint-related injuries. While initial diagnosis of such injuries is mainly performed by conventional imaging techniques — radiography, computed tomography (CT), and magnetic resonance imaging (MRI) — the detected tissue damage, such as a ligament tear, commonly requires repair intervention via arthroscopic surgery. During these surgeries, previously undetected tissue defects are often discovered because of the limitations of the conventional imaging techniques.
However, arthroscopic procedures rely on the use of conventional endoscopic cameras to visualize tissue surfaces, along with a metallic palpation hook to evaluate the tissue’s mechanical competence. These diagnostics tools are still the gold standard when deciding on the optimal treatment and, for example, choosing the amount of degenerated tissue to remove.
The proposed NIRS measurement system can be employed for diagnosis of any tissue where changes in tissue structure and composition are biomarkers of disease or degeneration. In the biophotonics research conducted at UEF, the focus is on optical characterization and diagnosis of connective tissues — articular cartilage, subchondral bone, the meniscus, and ligaments3,4,5. The main constituents of these tissues are water, collagen, and proteoglycans.
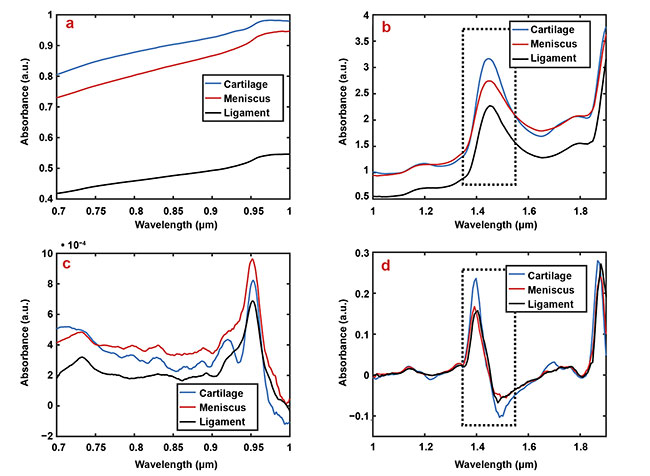
Figure 2. Representative smoothed (a, b) and first derivate (c, d) spectra of articular cartilage (blue), the meniscus (red), and ligaments (black).
For example, the relatively higher water content of cartilage compared to other tissues is apparent in subfigures b and d at the wavelength region
1.35 to 1.55 µm (dashed box). Courtesy of Biophysics of Bone and Cartilage/University of Eastern Finland.
The meniscus and ligaments have similar contents of water (65% to 75%) and collagen (20% to 25%), whereas cartilage has a relatively higher water content (80%) and lower collagen content (10% to 15%). In addition, ligaments have lower proteoglycan content (<1%) compared to articular cartilage and the meniscus (5%). These differences can be observed in certain spectral regions in the visible and near-IR spectra (Figure 2) and can provide detailed information on tissue properties (Figure 3). The spectrum has subtle and overlapping spectral features and because of this limitation, the applications of NIRS in medical applications are still scarce.
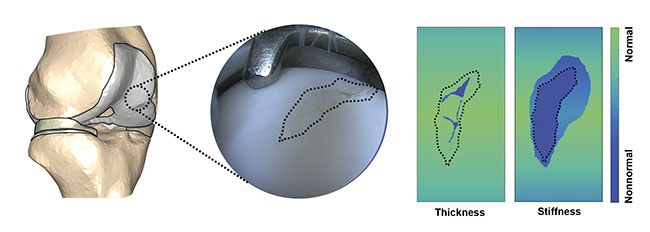
Figure 3. Spectral mapping of cartilage defect and surroundings to determine cartilage thickness and stiffness. The evaluation would provide surgeons with
information about the compromised or nonnormal tissue requiring surgical intervention. Courtesy of Biophysics of Bone and Cartilage/University of Eastern Finland.
To relate spectral changes to tissue properties, several mathematical approaches have been introduced. Previously, univariate analysis techniques, such as area under the curve (AUC), were used to estimate target properties. However, these techniques only use single wavelengths or narrow spectral regions, thereby omitting information from other possibly important spectral regions. In addition, such techniques fail to detect subtle changes in spectral features, which can only be extracted using derivative preprocessing.
Currently, principal component analysis (PCA) and partial least squares (PLS) are the most commonly applied analytical approaches for analyzing NIRS data in chemometrics. Both of these methods
construct new predictor variables (components) from linear combinations of the original predictor variables (different wavelengths of the near-IR spectra). PLS
is usually the more robust of the two,
as it accounts for the response variable (the tissue property) when determining the levels of variability in the predictor variables.
Lately, one-dimensional convolutional neural networks have been applied for spectral analysis, with promising results. The network structure conventionally includes an input layer, multiple hidden layers, and an output layer. Data processing happens in several hidden layers by weighting the inputs and processing them through various activations. The number of hidden layers has a substantial effect on the performance and computational time required for model optimization. First, hidden layers identify simple patterns (e.g., spectral peaks), while finer spectral features are extracted by the deeper layers.
Limitations and challenges
Despite these advancements in spectral analyses, the main limiting factor in the adaptation of NIRS in biomedical applications is associated with data availability. To reliably determine tissue properties, samples need to be extracted and subjected to time-consuming gold-standard laboratory measurements.
Unfortunately, the sample size in most NIRS studies is poor when compared to other studies that use state-of-the-art mathematical approaches, such as image classification applications. This limited sample size often leads to model overfitting, which results in poor predictive performance with new samples. Cross-validation (dividing data into subgroups) may improve the model’s generalizability without overfitting to the training data. With deep learning approaches, data
augmentation is often applied to artificially increase the size of data available for training. In image classification
problems, for example, the amount of data is increased by multiple factors by applying simple operations, such as image rotation. In spectral applications, the hardware enables swift subsequent measurements and, therefore, the collection of multiple spectra from the same location. Although, for these approaches, the best option is always to acquire more unique data.
While the NIRS technique has shown promise for applications in orthopedics,
several limiting factors need to be addressed prior to successful clinical adaptation. The previously described hardware-related issues are relatively easy to account for, whereas the process of optimizing spectral analysis to relate spectral changes to tissue properties is constantly developing. The penetration depth of near-IR light is known to be wavelength-dependent. However, no physical models currently exist to better understand the contributions of tissue constituents and structure to the measured spectrum.
Meet the author
Jaakko Sarin is a medical physicist trainee at Kuopio University Hospital in Finland. He has a doctorate in medical physics from the University of Eastern Finland, where he focused on optical spectroscopy of articular cartilage; www.researchgate.net/profile/jaakko_sarin.
Acknowledgments
The author would like to thank Jari
Torniainen, Isaac Afara, Juha Töyräs,
Juho Ala-Myllymäki, Harold Brommer, and Katariina Myller.
References
1. G. Spahn et al. (2009). How valid is the arthroscopic diagnosis of cartilage lesions? Results of an opinion survey among highly experienced arthroscopic surgeons. Archiv Orthop Unfall-Chir, Vol. 129, Issue 8,
pp. 1117-1121.
2. D. Chamberland et al. (2010). Optical imaging: new tools for arthritis. Integr Biol,
Vol. 2, Issue 10, pp. 496-509.
3. J.K. Sarin et al. (2018). Arthroscopic near-infrared spectroscopy enables simultaneous quantitative evaluation of articular cartilage and subchondral bone in vivo. Sci Rep,
Vol. 8, Article 13409.
4. J. Ala-Myllymäki et al. (2016). Optical spectroscopic determination of human meniscus composition. J Orthop Res, Vol. 34, Issue 2, pp. 270-278.
5. J. Torniainen et al. (2019). Near-infrared spectroscopic evaluation of ligament and tendon biomechanical properties. Ann Biomed Eng, Vol. 47, Issue 1, pp. 213-222.