Facial Recognition Technology Sees New Advances and Novel Applications
Novel technology and powerful new algorithms have facilitated a range of advances in face recognition systems, leading to improved accuracy in traditional applications – mostly in the areas of surveillance and security – and suggesting a host of new uses. By integrating these algorithms into social media platforms, for example, developers are taking “friending” and “tagging” to a whole new level.
In the real world – the nonvirtual world, anyway – face recognition systems can aid in security efforts by extracting faces from a photo or a live video feed and comparing them to reference images stored in a database. Every face has a number of distinguishable landmarks: the distance between the eyes, the width of the nose, the height and shape of the cheekbones, and so on. After measuring these landmarks, the systems generate a numerical code called a faceprint. This can then be digitally matched to the reference images.
Unfortunately, efforts to identify faces are generally confounded by differences in lighting, changes in face angles and a number of other factors. Face recognition systems have proved effective in controlled laboratory settings – with subjects looking directly into the camera and little variation in lighting, for instance – but real-world implementation has been more problematic.
Not surprisingly, there was a spike in interest in facial recognition in the months and years after the Sept. 11 terrorist attacks. Systems were installed in airports and other public areas with hopes of spotting individuals on terrorist watch lists, those suspected of other crimes and even runaway children. Tests at Logan International in Boston, as well as at airports in Dallas/Fort Worth, Fresno, Calif., and Palm Beach County, Fla., yielded disappointing results. In Tampa, Fla., police shut down a network of face recognition cameras because they had not produced any matches during a two-year trial period.
Work has ramped up considerably in the years since, however. “Recognition across variations in pose, lighting, expression, aging and occlusions have been studied for the last two decades,” said University of Maryland, College Park, graduate student Raghuraman Gopalan. Although, until the early 2000s, researchers generally addressed each of the concerns individually in the laboratory, “More recently there has been increased focus on unconstrained face recognition – when all these variations are present. This is primarily attributed to the proliferation of cameras in the real world.”
In a study reported in Computer Vision and Image Understanding earlier this year, Gopalan and University of Maryland, College Park, researcher David Jacobs tested the efficacy of a number of different algorithms in capturing face properties under varying lighting conditions. Importantly, they performed recognition with both homogeneous and heterogeneous lighting across subjects and with only one reference image subject. This made recognition a great deal more challenging, but it also better approximated the conditions of many real-world settings.
Thus they were able to determine the most effective of the algorithms for dealing with differences in lighting. “The primary contribution [of the study] is to show that a very simple method based on gradient orientation captures most of the person-specific information across lighting,” Gopalan said. “This can be combined with existing methods that handle other facial variations.”
Researchers have also sought to address these challenges using 3-D face recognition: A 3-D model of a face can be lit from any direction and can be rotated in any dimension to match the database model as closely as possible. Three-dimensional technologies, including stereo capture devices and structured light scanners, have been demonstrated successfully but can have drawbacks of their own.
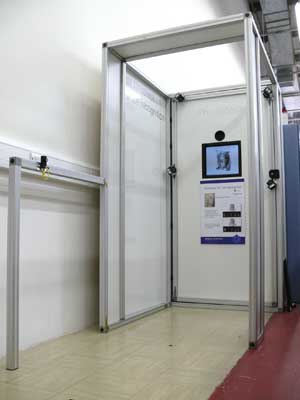
Researchers are developing novel approaches to 3-D face recognition, seeking to overcome obstacles posed by variations in lighting and face angle, for example. Shown here is a device developed by investigators at the University of the West of England and a 3-D model of a face (above right) captured using the device. Images courtesy of Machine Vision Laboratory, University of the West of England.
Mark F. Hansen, a PhD student at the University of the West of England in Bristol, elaborates: “The major disadvantages of 3-D recognition are the cost of capture devices and the fact that current devices require a great deal of interaction – for example, you must be positioned in a very narrow window – and so are not suited to nonintrusive access control systems.” At the same time, manipulation of 3-D data is computationally expensive compared with 2-D face recognition, while changes in expression still present a challenge for both 2- and 3-D technologies.
Various efforts are under way to address the shortcomings of 3-D face recognition technologies. At the University of the West of England, investigator Gary A. Atkinson has developed an approach called the PhotoFace 3-D capture device, which captures four images of a person under different illumination in about 20 ms. Based on these images, it estimates surface orientation using a technique called photometric stereo. The device offers a range of advantages, Hansen said, including the cost of building it, the time needed to acquire images, robustness against ambient illumination and more.
In another recent Computer Vision and Image Understanding study, Atkinson and Hansen, along with Lyndon N. Smith and Melvyn L. Smith, demonstrated the feasibility of deploying the device in real-world settings using either visible or near-infrared light. In fact, they found that using near-infrared light produced slightly more accurate reconstructions, which they attribute to subsurface scattering leading to a smoother skin surface.
The researchers continue to develop the technique. They have achieved high recognition rates on a subset of 1000 frontal and neutral expression sessions from the PhotoFace database and now hope to extend this capability to deal with changes in pose and expression. “Human beings are extremely good at recognizing familiar faces and seemingly suffer less from the same problems of expression, illumination and pose variance than automated systems,” Hansen said. “We are currently looking into ways that we can incorporate human-based heuristics into the algorithms.”
Ultimately, they envisage use of the device in border control, building access and situations in which automated access control is required.
Friending, tagging, tracking
We are now seeing face recognition technology in a host of other applications, including a number of social media tools. One of the next-generation Google Android phones, for instance, offers a handy face recognition feature. When you tag photos of friends, the phone will recognize the friends in other images and associate them with the appropriate contact information. Thus you will be able to call, Facebook and tweet your friends by touching them in photos on your phone.
Another Android-based application allows you to gather public information about people simply by pointing your phone at them. When you do so, Facebook, YouTube and Skype icons – among others – will pop up, enabling you to add them as friends, watch videos they have uploaded and so on. Those concerned about privacy need not worry: The application works only with users who have opted in.
We don’t always need to know who we’re looking at. With some face recognition applications – those being developed for digital cameras, for instance – it’s enough to know that we’re seeing a face at all and where it is in the image.
One example: The FotoNation FaceTracker solution from Tessera uses a heuristically based statistical algorithm to find “candidate” faces in images recorded by digital cameras and then to track them while confirming that the subject is in fact a face.
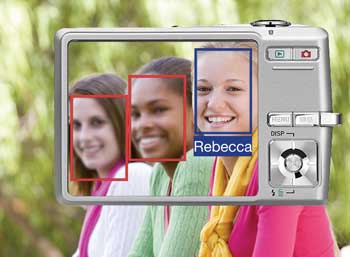
Face recognition is finding new uses beyond surveillance and security – thus far the primary applications of the technology. For example, complex face recognition algorithms now can find and track human faces in photos captured by digital cameras – helping to improve image quality correction with respect to faces in particular. Courtesy of Tessera.
Eran Steinberg, CTO of the Imaging & Optics Div. at the San Jose, Calif.-based company, explained that the solution was developed in response to analyses of human picture-taking behavior and human psychology when viewing images. Studies have shown that the overwhelming majority of photos taken by consumers include a human being; furthermore, they have revealed the very human tendency when viewing photos to focus first on any faces and only after this to investigate the rest of the image.
Given this, Steinberg said, “It makes sense to have the human faces come out best in an image and, in many cases, have the faces drive the overall image quality correction of the captured images. So, it stands to reason that finding and tracking the faces in the images enables camera developers to improve the quality and the automated decision of how best to capture and improve pictures of, for example, their friends and families.”