Mark Clymer1 and Dr. Eduardo Rosa-Molinar2,3
1Datacolor Inc., Lawrenceville, NJ 08648 USA
2Biological Imaging Group, University of Puerto Rico-Río Piedras, San Juan
3Institute of Neurobiology, University of Puerto Rico-Medical Sciences, Old San Juan
Establishing a standard could allow microscopists to verify and reproduce results.
Laboratory managers and researchers generally agree that having more accuracy and consistency in color rendition could assist in achieving improved evaluation of results. However, color management has seemed so complex and elusive that many scientists and medical professionals have given up on achieving it.
Transmitted-light bright-field microscope images have an enormous number of variables outside the specimen itself, all of which contribute to the creation of color in the final image. Results vary not only because of the microscope and camera used to capture them, but also because of such accessories as lamp houses, filters and image-acquisition software. Changing the intensity of, or voltage to, a halogen lamp, for example, changes the color of its output and ultimately impacts the color of the final image. Similarly, cameras are intended to provide an objective image for viewing, but there is no standard among manufacturers with regard to color adjustments being made by the imaging device.
Although digital imaging has been a boon to scientists for purposes of documenting their research with much greater ease than film-based photomicrography, it has also created an environment in which color varies more widely due to camera quality and acquisition software. In addition, monitors and other displays add further variability as to how images are perceived.
Despite the growing demand for standards that assure consistency in comparing and evaluating results in science and medicine, and an increasing focus on scientific integrity in imaging, color accuracy continues to be an issue. For example, an Internet search for the term “histology” reveals a disturbing variation in the color of images presented for scientific and medical purposes. Similar results are
seen in scientific poster presentations and in images found in respected science magazines. Key hues range from red to pale pink, purple, brown, blackish, rose, magenta and violet. These enormous color discrepancies may result in a degradation of perceptible detail and have a negative effect on interpretation and analysis.
Scientists seeking color consistency in images have relied on software programs such as Photoshop. However, “these technologies are cheap and easy to use, but also – for the panicky or unscrupulous – tempting to abuse,” according to the director of the Division of Investigative Oversight in the Office of Research Integrity at the U.S. Department of Health and Human Services.1
Unfortunately, with the changing landscape of imaging technology, many researchers have only a hazy understanding of acceptable and unacceptable types of individual alterations. For example, using software to enhance the overall clarity of an image is generally acceptable, but altering particular parts of an image or inserting data/features that affect the results or change the likely interpretation is not.
Many microscope users have been adjusting images for legitimate reasons. For example, microscopists may take hours white-balancing their images or using Photoshop or other software to highlight visible data. However, because such adjustments are performed using subjective measures of color accuracy – eyes and memory, more often than not – images from one session to another are unlikely to be comparable. Furthermore, there is little control to the degree of adjustment that may be applied to an image, potentially leading to the creation and reporting of artifacts.
Despite the calls for standards in science and despite the acknowledged benefits of consistent and accurate color for images used in science and medicine, it has been difficult for microscopists to establish a consistent color baseline for either acquisition or analysis.
A standard for color rendition that preserves original image data and is automatically applied across a set of images will assist in attaining maximum results while avoiding individually applied algorithms that might be questioned. And a new system could represent a step toward such a standard. ChromaCal from Datacolor Inc. works by capturing a color fingerprint of the entire imaging session and creating a color-correction algorithm; then, it automatically color-calibrates each specimen image. It also automatically white-balances and matches brightness, thereby further improving image color accuracy and consistency (Figure 1). The original image data is preserved for future use.
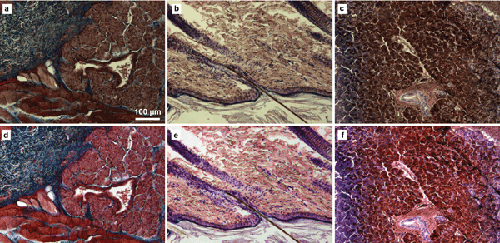
Figure 1. Three image pairs demonstrate how color calibration normalizes divergent images in accordance with consistent color standards and may help scientists discern important image detail. The images in the top row are ‘before’ images – raw images as rendered by Photoshop or MS Office software. In the bottom row are ‘after’ images – the same images after color standardization using Datacolor ChromaCal. Top and bottom left (a and d) are rabbit tongue, Masson’s trichrome stain; top and bottom center (b and e) are skin hair follicles, hematoxylin and eosin (H&E) stain; top and bottom right (c and f) are pancreas, silver stain. Images courtesy of Datacolor Inc.
Users capture a series of specimen images as usual. During the same session, they capture one additional image of the color-calibration slide that is engineered to cover the full dynamic range and contains known color properties. The system’s software then compares the color-calibration image to the known values and automatically generates a color-calibration profile. This profile is used to create calibrated versions of each specimen image in the session. The original image and its newly calibrated version are presented on-screen for comparison. To provide a complete audit trail, the calibrated image is saved separately from the original image file and includes documentation of the calibration process in its metadata.
Calibration after the image-capture process is an important factor in attaining image consistency, but it is not the only factor. As anyone who has ever shopped for a television or computer knows, the same images are inconsistent when shown on different monitors. Users’ ability to adjust monitors to their taste or standards does not ensure calibration to any real standard. Even worse, it is possible that some details containing critical information could be lost in translation from original image to uncalibrated display output. In fact, scientists rarely empirically calibrate their monitors, even though this is common practice in color-centric industries such as photography, textiles, paints and finishes, where acquisition/measurement devices and output/production devices are all calibrated to deliver a standardized, reproducible product. An additional factor related to image inconsistency is that a monitor’s ability to display colors deteriorates over time. Thus, an issue that must be addressed is the way images appear when viewed by laboratory supervisors, colleagues, consulting physicians or fellow researchers across the lab or around the globe.
To address variation and other monitor issues, the new system uses a colorimeter to objectively measure color output from the monitor, and the software returns the monitor display profile to an industry standard (i.e., sRGB). If every monitor in a laboratory is calibrated, every user will be able to see images that adhere as
closely as possible to a single standard for color. Even in fluorescence imaging, a calibrated monitor can deliver greater tonal ranges to reveal finer specimen detail. Monitor calibration assists in improving the quality of slide review – and, therefore, of teaching, training and eliciting second opinions, among other functions.
With little consistency among imaging systems today, the development of color-management standards for research imaging is highly desirable. When scientists eliminate unpredictable variation that occurs during image capture and display, they reduce an unwanted variable in their experiments and analyses. Color calibration in bright-field microscopy is becoming a vital step in this process.
Meet the authors
Mark Clymer is the director of marketing for Datacolor Scientific at Datacolor Inc. in Lawrenceville, N.J.; email: [email protected]. Dr. Eduardo Rosa-Molinar is the group leader of the Biological Imaging Group at the University of Puerto Rico-Río Piedras in San Juan and associate professor of neurobiology (adjunct) in the Institute of Neurobiology at the University of Puerto Rico-Medical Sciences in Old San Juan; email: [email protected].
Reference
1. M. Hendricks (January 2011). “Scientific Integrity in the Age of Photoshop,” Johns Hopkins Medicine, Institute for Basic Biomedical Sciences, news article. www.hopkinsmedicine.org/institute_basic_biomedical_sciences/news_events/articles_and_stories/employment/2011_01_scientific_integrity.html.
Color science in black and white
Color perception is caused by a combination of a light source, an object and human vision. The illumination source emits light with a particular intensity distribution over the visible wavelength range. An object intercepts some of this light, absorbs different amounts at various wavelengths, and reflects or transmits the rest. Human eyes then receive the reflected/transmitted light and send signals to the brain. The brain processes these signals and finally conveys the color sensation. Colorimetry is the science and technology of quantifying color and predicting perceptual color matches based on physical measurements. Its goal is to reduce the variation that can creep in via image capture (cameras), rendering (display) and human interpretation (the eye and brain).
A typical digital color camera has three color channels. Each pixel of most color images can be assigned three values: R, G and B, representing the red, green and blue parts of the light spectrum that the camera perceives. However, colors that the camera conveys can be quite different from those seen by a human (e.g., scene purples may appear blue in a camera image). Part of the problem is that, with almost any camera, colors that the camera portrays as identical may not be a color match to the human visual system. This problem is caused by the mismatch between the spectral sensitivity of the RGB pixels in cameras and that of the cone cells in human eyes. Even worse, individual camera brands have different spectral sensitivities, making colors from various cameras look quite different.
Image rendering is another source of inconsistency. A typical color-rendering device, such as a computer monitor or display, relies on the mixing of key primary colors to render the displayed colors. Because of the limited number of primaries used by any individual monitor, the spectrum of the rendered color never completely matches the reflected-light spectrum of the original object. Different monitor brands use different primaries and different mixing recipes, which results in different displayed colors, even with identical input.
Human interpretation is another area of inconsistency. People distinguish colors due to photosensitive cone cells on the retina. There are three types of cones, with spectral sensitivity peaked at different wavelengths, allowing human eyes to separate light into red, green and blue channels. However, all people do not have the same spectral cone responses. People sometimes see color differently even if the light source and the object are the same, making color quantification even more difficult.
In 1931, the CIE (the International Commission on Illumination) defined the standard (colorimetric) observer to represent an average human’s chromatic response, with the numerical description being defined as the following color-matching functions: ‾ x (λ), ‾y(λ) and ‾z (λ), where λ is wavelength. CIE further defined a model that, for a particular object under a particular light source, allowed the calculation of standard tristimulus values (X-Y-Z) that are related to the cone responses of the eye to that object and light. X-Y-Z tristimulus values objectively quantify the color of a particular object under a particular light source and are at the heart of color standardization.
To address inconsistencies, a series of calibrations and conversions is required along the color process pipeline, from color capture all the way to color rendering. The technology used for such color calibration and conversion is called color management. Although camera and monitor display vendors can have their own proprietary color calibration algorithms, the International Color Consortium (ICC) has recommended the adoption of a standard color management system architecture and components called ICC Profile Specification (ISO 15076-1:2005), which can work across different platforms and devices. Such specification provides a framework for color calibration of individual devices and subsequent communication among calibrated devices.
For more information, visit www.color.org and www.color.org/specification/ICC1v43_2010-12.pdf.
– Dr. Hong Wei, Datacolor Inc.