In early studies, a fast, nondestructive technique allows for the differentiation of patients with Alzheimer’s disease from healthy individuals and patients with other types of dementia.
NICOLE RALBOVSKY AND IGOR K. LEDNEV, UNIVERSITY AT ALBANY, SUNY
Raman hyperspectroscopy combined with advanced statistics is uniquely suitable for characterizing microheterogeneous systems. Understanding the structure and biochemical composition of samples at the microscopic level is important for many practical applications, including various bioanalytical tasks.
The great potential of Raman hyperspectroscopy for medical diagnostics is based on its ability to integrate the impact of multiple biomarkers in a specific spectroscopic signature. This approach was used for the development of a minimally invasive method for diagnosing Alzheimer’s disease (AD) based on blood analysis. Multivariate data investigation of blood serum Raman spectra allows for the differentiation of patients with AD from those with other types of dementia and from healthy individuals.
AD is a neurodegenerative disorder characterized by progressive cognitive decline, eventually leading to death. It is the most common form of dementia among individuals over age 65 and is the sixth leading cause of death in the United States, with no cure available. According to the Alzheimer’s Association, 5 million Americans are affected by AD, and this number is anticipated to triple by the year 2050. Treating Alzheimer’s disease is difficult because there is no way to definitively diagnose it. Only post-mortem examination of the brain can confirm presence of the disease. Raman hyperspectroscopy can change that.
Hyperspectral imaging obtains a spectrum for each individual pixel of an image taken of a sample for the purpose of determining the spatial distribution of components within it. A typical experiment generates a massive data set called a hyperspectral data cube, which consists of two (or three) spatial coordinates and a spectrum. Various spectroscopic techniques have been used for hyperspectral imaging, including fluorescence and UV-VIS and IR absorption.
Raman spectroscopy has shown significant promise for hyperspectral imaging because of its exceptional chemical specificity. Raman spectroscopy, in general, is a fast and nondestructive technique that produces a specific spectroscopic fingerprint of the material studied. The method involves irradiating a sample with monochromatic light. This irradiation causes the molecules in the sample to vibrate, producing shifts in energy of the scattered photons.
The energy shifts result in a characteristic Raman spectrum, with individual peaks corresponding to a particular vibrational mode. All biochemical components of the sample contribute to the Raman spectrum proportionally to their concentration and inelastic light-scattering efficiency. With such detailed spectra produced, minute differences in composition can be illuminated when comparing two different samples, such as healthy and diseased tissue.
Raman hyperspectroscopy generates a hyperspectral data cube, with a focus on determining spectral components and consequently the biochemical composition of the sample. Notably, the average spectra of different classes of data are often visually similar — this is because averaging a class of data causes the specific, but minute, differences in individual spectra to be lost, resulting in otherwise distinct classes of data to appear similar to each other (Figure 1).
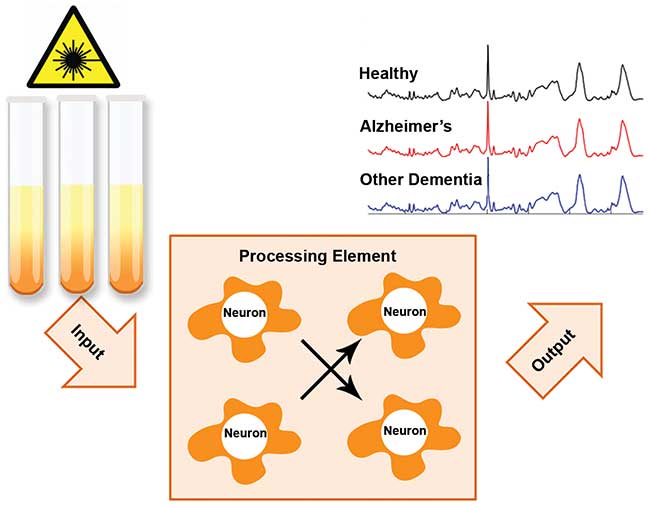
Figure 1. Multivariate statistical analysis of Raman hyperspectral data sets obtained for blood serum samples can be used to differentiate Alzheimer’s disease patients from healthy people and patients with other forms of dementia, even though the average class spectra appear to be similar to the naked eye. This technique can be used as a method for diagnosing Alzheimer’s disease. Adapted with permission from reference 4.
However, the analysis of hyperspectral data cubes acquired for different classes of data can be improved through the use of advanced statistical analysis, or chemometrics. Chemometrics uses mathematical and statistical methods to extract information from complex analytical data; it takes into consideration individual spectral data, without averaging, and thus capitalizes on minute differences for the purpose of classification. The combination of hyperspectroscopy and advanced statistics has powerful analytical applications, including disease diagnostics.
A typical approach for developing a new diagnostic method for a specific disease involves three steps (Figure 2, top row). First, researchers choose a biomarker specific to the disease. Next, they develop a bioanalytical tool to determine the presence of the biomarker and/or quantify it. Finally, they conduct a pilot clinical trial to evaluate method selectivity and sensitivity.
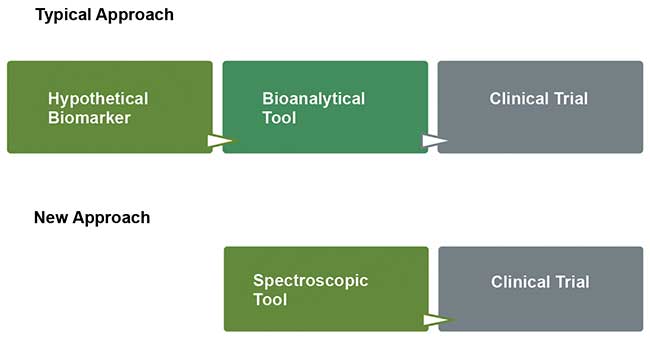
Figure 2. Typical approaches to diagnosing diseases involve three lengthy steps (top row). A two-step approach using Raman hyperspectroscopy can save time and money (bottom row). Courtesy of Nicole Ralbovsky.
Misfolded amyloid-beta peptide in a β-sheet-rich conformation is considered a biomarker of AD. Attenuated total reflection Fourier transform IR (ATR-FTIR) spectroscopy, which is another vibrational spectroscopy technique, has recently been used for detecting amyloid-beta peptide in patients’ blood1. The study reported 84 percent accuracy for differentiating AD patients from healthy people.
A new diagnostic method consists of only two steps, but obtains better statistical results (Figure 2, bottom row). The method characterizes samples as belonging to a certain donor class by probing the total biochemical composition with Raman hyperspectroscopy. Advanced statistical analysis of the acquired spectral data set builds a classification model, which can differentiate classes.
Multiple biochemical markers could contribute to the spectroscopic characteristics that allow for class differentiation, including markers that are not yet considered by the scientific community for the specific disease. Probing the entire biomedical composition dramatically increases specificity. It also saves a large amount of time and effort for determining potential biomarkers.
This new approach results in greater accuracy than conventional methods, while exploiting multiple, potentially undiscovered biomarkers for a disease. Initial research into this method used Raman hyperspectroscopy to differentiate blood samples taken from healthy donors from those with AD and other forms of dementia.
Current diagnostic efforts for AD involve a lengthy and arduous combination of tests and examinations. Variations of mental health and mood exams as well as physical, neurological, and imaging tests are among those used. Although a combination of these tests can lead to a “loose” diagnosis, there are considerable drawbacks. Cerebrospinal fluid analysis is invasive, requiring a lumbar puncture. The Mini-Mental State Examination is subjective. Imaging tests are expensive and work only to eliminate other potential diseases.
The initiation of disease-modifying treatments at the earliest stages of AD pathogenesis might be the most effective way to treat it. But this requires that patients are identified as having AD as early as possible, before neurodegeneration becomes too severe2. There are several medications on the market that can be prescribed to a patient thought to have AD. However, these medications only work to lessen symptoms, and they have serious side effects, making prescribing them less appealing when a diagnosis is not certain.
Because Raman hyperspectroscopy is so specific, it can monitor the changes in biological composition as a disease progresses. When chemometric techniques are used in combination with the highly specific and complex spectral data cubes produced by Raman hyperspectroscopy, a robust method for diagnosing AD begins to emerge.
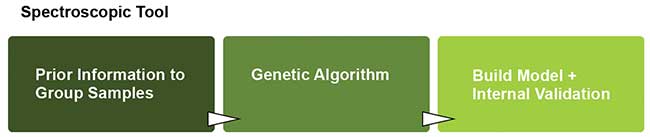
Figure 3. In this workflow of a spectroscopic tool, data cubes of samples, with known class assignments, are uploaded into the chemometric software. Genetic algorithm (GA) is used to identify the most significant spectral data for differentiation. Artificial neural network (ANN) and support vector machine discriminant analysis (SVMDA) chemometric models are built and undergo internal validation. Courtesy of Igor Lednev.
A spectroscopic tool designed to do this collected 121 spectra from each of 48 samples, according to a simple schedule (Figure 3). In total, 5808 spectra were uploaded into MATLAB R2012a software and labeled with their respective class. A chemometric technique called genetic algorithm (GA) picked out the most statistically relevant sections of the Raman spectra for differentiating the classes from each other (Figure 4).
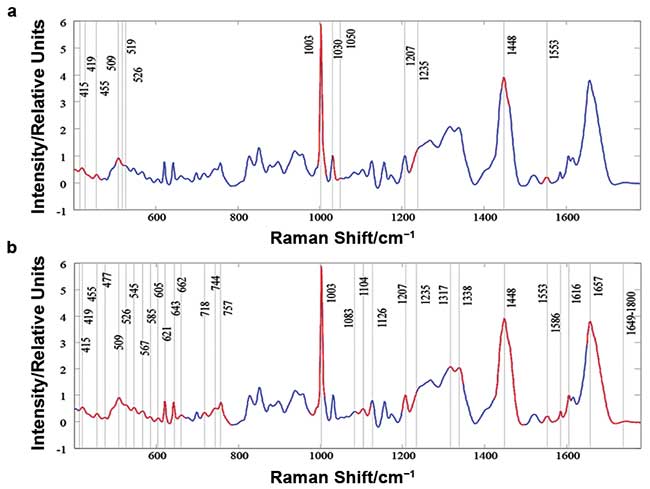
Figure 4. Genetic algorithm (GA) analysis: Highlighted spectra (red) show the most significant regions of the Raman spectra used for differentiating Alzheimer’s disease patients from healthy controls (a) and other forms of dementia (b). Adapted with permission from reference 4.
The majority of the bands are assigned to biomolecules previously reported as being potential AD biomarkers. Several of the highlighted (in red, Figure 4) spectroscopic markers correspond to phospholipids and metabolites, such as phosphatidylinositol, phosphatidylcholine, proline, lysine, and phenylalanine, all of which have been validated as part of an AD biomarker panel3,4.
After GA analysis, two different modeling techniques were assessed to see which would produce the best classification results.
The first technique is support vector machine discriminant analysis (SVMDA), which used known information as a training data set (Figure 5, left panel). The model is told to which class each sample belongs, and it learns how to group classes together based on spectral regions with high discriminatory power, identified through GA. Every one of 121 spectra collected from each of the AD and healthy control samples are plotted as its own point. As expected, each spectra from moderate AD samples (red triangles) shows 100 percent probability of being an AD sample, whereas the healthy controls (blue area) show a zero percent probability.
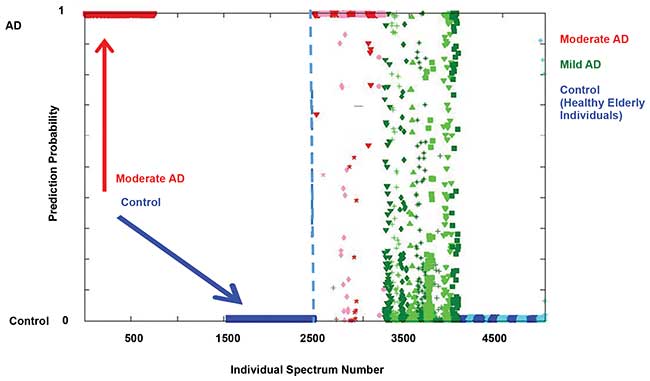
Figure 5. The left panel of the results of support vector machine discriminant analysis (SVMDA) classification is the training data set, and the right panel is the validation set. Results show an average sensitivity of 86 percent and specificity of 93 percent for differentiating the three classes. Courtesy of Igor Lednev.
The next step is internal validation. Here, leave-one-out cross-validation builds the model with spectra from all samples but one. The 121 spectra from the sample that was not used for building the model are individually used to test how the model makes predictions on an unknown sample. This process is repeated until each sample has been left out, and the results are shown in Figure 5, right panel. Each sample is separated from another of the same class by slight differences in color (e.g., pink versus red triangles for moderate AD).
The technique correctly predicts the majority of spectra from both the healthy and moderate AD classes. Although some single spectra for moderate AD and healthy controls have varying probability, the prediction for each of these samples as an average of the 121 assignments is correct. Interestingly, there is a wide probability distribution for the mild AD samples (green squares). This shows the high sensitivity of Raman hyperspectroscopy.
Biomarkers can be present at incredibly small concentrations in body fluids, such as blood serum, as is the case with most proteins and biological components. The Raman hyperspectroscopy method takes multiple spectra per sample. Some of these may resemble a healthy spectra because biomarkers are not present in that particular spot of the sample. This is expected because of the heterogeneous environment of the body fluid.
There also will be spectra that show a strong presence of detected biomarkers. The result is a disbursed probability for the mild AD patients. The samples as a whole are not completely healthy, yet not totally considered moderate AD cases. This is enough to consider a sample as affected by AD, but at an earlier stage of disease progression. The SVMDA classification model presented has an average sensitivity of 86 percent and specificity of 93 percent for differentiating the three classes.
An artificial neural network (ANN) was built in order to improve on these results. ANNs are simplified mathematical models that resemble the human brain. They are made up of several layers of simple interconnected processing elements called neurons. Each neuron can perform a mathematical operation on input values and transfer the result to one or more hidden layers or to an output layer (Figure 6). The connection between the different neurons takes the information obtained through GA and uses it for classification. ANNs are used to differentiate patients with mild and moderate AD from healthy people. The results showed an average sensitivity of 93 percent and specificity of 94 percent, a significant improvement over the SVMDA model. The high levels of sensitivity and specificity4 show how successful Raman hyperspectroscopy combined with chemometrics can be for diagnosing AD.
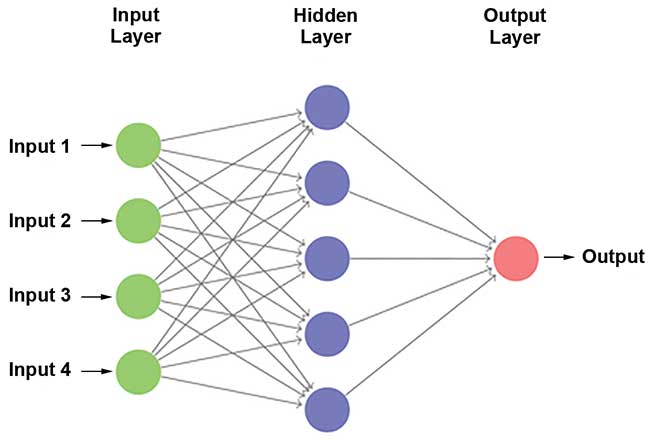
Figure 6. General scheme for an artificial neural network (ANN) model technique. Courtesy of Nicole Ralbovsky.
The next step for this research is advanced method development. This will involve a significantly larger number of samples to establish the method specificity and sensitivity for a much wider variety of donors.
Raman hyperspectroscopy, combined with advanced statistical analysis, has a huge potential to be used for disease diagnostics. It also shows extreme promise for any other disease-exhibiting physiological changes as its pathology progresses and for applications in the field of forensic science5-11.
Meet the authors
Nicole Ralbovsky is a graduate student at the University at Albany, SUNY. She holds an ACS-certified bachelor of science degree in chemistry from SUNY Geneseo. Her research focuses on Raman hyperspectroscopy and its applications for disease diagnostics; email: [email protected].
Igor K. Lednev is a professor at the University at Albany, SUNY. His research is focused on the development and application of laser spectroscopy for biomedical and forensic purposes. He is a fellow of the Society for Applied Spectroscopy and has co-authored over 200 publications in peer-reviewed journals; email: [email protected].
References
1. A. Nabers et al. (2016). Amyloid-β-secondary structure distribution in cerebrospinal fluid and blood measured by an immuno-infrared-sensor: a biomarker candidate for Alzheimer’s disease. Anal Chem, Vol. 88, Issue 5, pp. 2755-2762.
2. H.H. Kaj Blennow et al. (2010). Cerebrospinal fluid and plasma biomarkers in Alzheimer disease. Nat Rev Neurol, Vol. 6, Issue 3, pp. 131-144.
3. M. Mapstone et al. (2014). Plasma phospholipids identify antecedent memory impairment in older adults. Nat Med, Vol. 20,
Issue 4, p. 415-418.
4. E. Ryzhikova et al. (2015). Raman spectroscopy of blood serum for Alzheimer’s disease diagnostics: specificity relative to other types of dementia. J Biophotonics, Vol. 8, Issue 7, pp. 584-596.
5. C.K. Muro et al. (2016). Forensic body fluid identification and differentiation by Raman spectroscopy. Forensic Chem, Vol. 1, pp. 31-38.
6. A. Sikirzhytskaya et al. (2017). Determining gender by Raman spectroscopy of a bloodstain. Anal Chem, Vol. 89, Issue 3,
pp. 1486-1492.
7. C.K. Muro et al. (2016). Sex determination based on Raman spectroscopy of saliva traces for forensic purposes. Anal Chem, Vol. 88, Issue 24, pp. 12489-12493.
8. C.K. Muro and I.K. Lednev (2017). Race differentiation based on Raman spectroscopy of semen traces for forensic purposes. Anal Chem, Vol. 89, Issue 8, pp. 4344-4348.
9. E. Mistek et al. (2016). Race differentiation by Raman spectroscopy of a bloodstain for forensic purposes. Anal Chem, Vol. 88, Issue 15, pp. 7453-7456.
10. G. McLaughlin et al. (2014). Raman spectroscopy of blood for species identification. Anal Chem, Vol. 86, Issue 23, pp. 11628-11633.
11. K.C. Doty et al. (2017). Predicting the time of the crime: bloodstain aging estimation for up to two years. Forensic Chem, Vol. 5, pp. 1-7.