Due to its label-free functionality, multiphoton microscopy accompanied by machine learning could soon complement traditional fluorescence imaging in the treatment of pancreatic cancer.
Noelle Daigle, Shuyuan Guan, and Travis Sawyer, University of Arizona
Pancreatic cancer is one of the deadliest malignancies, with an average five-year survival rate of only 12%1. Surgical resection of the tumor is often the only realistic approach to saving a pancreatic cancer patient, but only if the malignant tissue can be completely removed. An incomplete resection results in the cancer recurring or metastasizing, which typically leads to the death of the patient. The current standard of care for assessing the completeness of resection is pathological inspection of resected tissues for defining “margins.” Multiphoton microscopy (MPM) has the potential to enable this assessment at the point of care, facilitating more rapid and complete treatment of the disease.
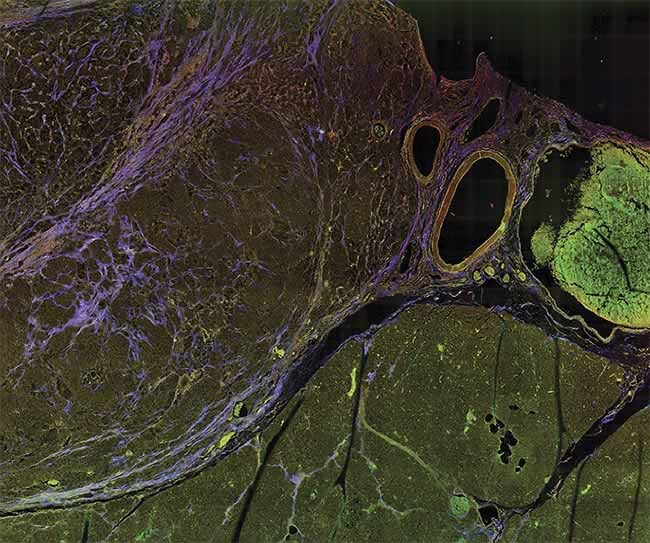
A multiphoton microscopy (MPM) image of tissue, showing striking contrast for identifying margins. Courtesy of University of Arizona.
Surgical resection of the tumor is often the only realistic approach to saving a pancreatic cancer patient, but only if the malignant tissue can be completely removed.
A positive margin is identified when the cancer reaches the edge of the resected tissue, indicating that there is residual cancer in the patient, whereas a negative or “clear” margin implies a complete resection (Figure 1). However, there are major shortcomings in traditional pathological inspection that limit its utility during surgery. For example, this approach involves preserving and then cutting resected tissues into thin sections and inspecting them under a high-magnification microscope for the presence of cancer cells. While this technique is widely considered to be the gold standard in cancer diagnostics, this only enables sampling and inspection of a very small volume of the tissue. This limitation can lead to physicians overlooking positive margins, particularly for cancers that are more diffuse in nature. Furthermore, the delay required for conducting this process can be lengthy and inhibits true intraoperative action when it is most effective.
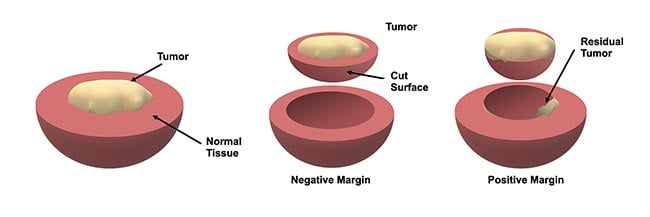
Figure 1. An illustration of how margins are defined during tumor resection. Typically, the resected tissue is evaluated by a pathologist to assess whether the tumor reaches the boundary, which implies that there may be some residual tumor in the patient — this is known as a positive margin. Courtesy of University of Arizona.
Sharpening the image
Consequently, techniques to accurately visualize tissue in real time during surgery could greatly improve rates of complete resection as well as reduce procedure times and minimize excess tissue removal. If this result is achieved, it could improve both patient prognosis and overall morbidity.
Unfortunately, current methods for intraoperative guidance during pancreatic cancer surgery are not suited for margin definition. Fluorescence imaging with a tumor-targeted contrast agent is commonly used for surgical guidance but relies on an abundance of tumor cells to express the fluorescent agent; therefore, for residual margins, the signal level can be decreased to undetectable levels. Moreover, instrumentation for fluorescence-guided surgery, often incorporating low-magnification imaging lenses, is designed for a large field of view and relatively low resolution, which prevent resolving small-scale features. Similarly, intraoperative ultrasound is commonly used for tumor localization and has the advantage of providing depth information, but the resolution of this technique is insufficient for assessing margins at the microscopic scale.
Therefore, while current methods are useful for localizing tumor bulk prior to resection, there is a significant unmet need for intraoperative margin definition. Pancreatic cancer exhibits unique microstructural and molecular features that could be leveraged to provide high contrast for defining margins intraoperatively. One such feature is the strong stromal (connective tissue) reaction that produces aberrant collagen networks in, and around, the tumor. Additionally, pancreatic cancer cells can naturally produce molecular products related to cellular metabolism and senescence that provide a unique fluorescent signature. The authors’ research at the University of Arizona aims to target these signatures by combining advanced two-photon imaging technology with machine learning classification to provide real-time surgical guidance that could bridge the gap to enabling intraoperative margin definition.
Visualizing intrinsic biomarkers
These results are extremely promising for showing the potential of two-photon microscopy coupled with
deep learning for providing real-time guidance for tumor margin definition during a variety of cancer surgeries.
MPM is a label-free imaging technique capable of visualizing tissue at the cellular level, with several advantages for live imaging compared to standard single-photon techniques, such as light microscopy and confocal microscopy2. MPM uses multiple-photon excitation, wherein the sample is excited by two or more photons of the same wavelength. These photons must be nearly simultaneously incident at the same volume of the tissue to produce fluorescence, resulting in high resolution in all three spatial dimensions. To deliver the same amount of energy as in light microscopy, incident light must be 2× or 3× the wavelength, which is usual in the case of two- and three-photon excitation, respectively (Figure 2a).
Consequently, MPM uses long, near-infrared wavelengths, which have greater penetration depth in tissue and cause less photodamage than visible light. Because of these characteristics, MPM has gained popularity within the last several decades as a noninvasive, high-resolution imaging modality in biomedical fields, including oncology, immunology, neuroscience, and cardiovascular studies. And MPM may soon provide vital life-saving detail in the intraoperative margin definition of pancreatic cancer.
To apply MPM for intraoperative margin definition of pancreatic cancer, the authors’ team focused on collecting three wavelength-resolved imaging channels, which were selected to probe two endogenous fluorophores and second harmonic generation (SHG), a nonfluorescent nonlinear optical response related to molecular structure and polarization predominantly produced by collagen. The two fluorophores targeted were nicotinamide adenine dinucleotide and hydrogen (NADH) and lipofuscin3. NADH is connected to cellular metabolic processes, and lipofuscin has been associated with cellular senescence. These autofluorescent molecules and SHG represent avenues to probe dysregulation on a cellular and structural level as a result of cancer.
By selecting the appropriate excitation and emission wavelengths to best separate each channel from one another, the signal from each fluorophore can be quantified, spatially resolved, and used to inform a deep learning algorithm for automated image classification (Figure 2b). This ultimately provided real-time intraoperative guidance in margin definition for surgeons such as collaborators Mohammad Khreiss, M.D., at Banner-University Medical Center and Naruhiko Ikoma, M.D., at the University of Texas MD Anderson Cancer Center.
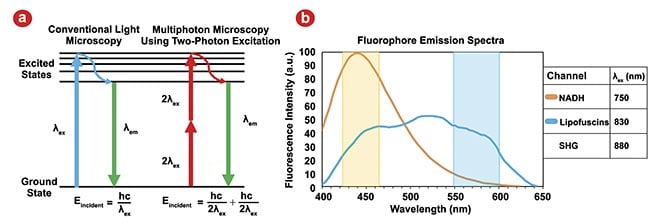
Figure 2. Traditional microscopy using single photon excitation contrasted with multiphoton microscopy (MPM) using two-photon excitation. Eincident: energy incident on the sample; λex: excitation wavelength; λem: emission wavelength. Adapted with permission from Reference 2 (a). Emission spectra for the two endogenous fluorophores for an MPM excitation wavelength of 732 nm; second harmonic generation (SHG) will generate emissions at λex/2. Actual excitation wavelengths used for all MPM channels are listed in the legend, and colored overlays correspond to our detection wavelength ranges for each channel. The signal from each channel is dominated by its fluorophore, but some overlap is unavoidable. NADH: nicotinamide adenine dinucleotide and hydrogen. Adapted with permission from Reference 3 (b).
Real-time image classification
Under the larger umbrella of deep learning, convolutional neural networks (CNNs) have emerged as a powerful tool for automatic image classification, revolutionizing industries from defense and security to medical imaging. The architecture of CNNs is composed of a sequence of programmable layers, each playing a vital role in extracting quantitative features from image data.
Figure 3 shows the general architecture of a CNN. At the heart of CNNs are convolutional layers, designed to extract essential image features, such as edges and textures, laying the foundation for employing this information for a specific task such as classification. After each convolution operation, an activation function introduces nonlinearity into the model, allowing it to learn more complex patterns. The Rectified Linear Unit is the most commonly used activation function in CNNs, which replaces all negative pixel values in the feature map with zero. Pooling layers are introduced in this sequence, strategically reducing spatial dimensions and computational demands while capturing dominant, multi-scale features that are essential for accurate analysis.
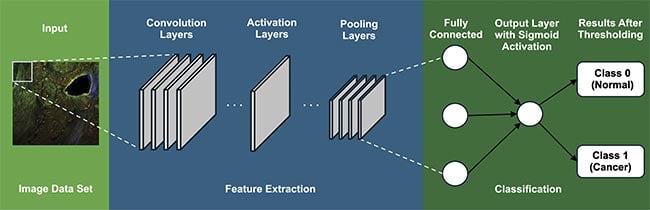
Figure 3. A general architecture of convolutional neural networks (CNNs), which involve a series of layers. Courtesy of University of Arizona.
Additionally, fully connected layers feature “neurons” that connect to all activations in the previous layer, crucial for high-level reasoning and decision-making in the network. Often in classification tasks, Softmax or sigmoid layers are employed to convert the network’s output into a probability distribution, indicative of the likelihood of the input belonging to a particular class4. These elements converge to empower CNNs with unparalleled capabilities for processing, analyzing, and learning from imaging data.
CNNs automatically learn to extract the most information-dense image features through training, obviating the need for manual extraction, in stark contrast to traditional methodologies. In biomedical image analysis, CNNs can achieve particularly high performance through the implementation of transfer learning, which is a method in which models pretrained on extensive data sets seamlessly adapt as feature extractors for new, data-limited tasks. This approach accelerates training on novel endeavors by repurposing knowledge gleaned from previous tasks.
Current research aims to implement cutting-edge CNNs for classifying MPM images of pancreatic cancer to yield real-time intraoperative guidance. However, the challenge was the lack of extensive data sets specific to this application, coupled with the impracticality of generating a large volume of data. To circumvent this limitation, four pretrained CNN architectures were strategically employed: VGG16, ResNet50, EfficientNet, and MobileNet. These models were selected due to their availability in the open-source Keras application programming interface and their pretraining on the ImageNet Large Scale Visual Recognition Challenge data set. These models span a wide range of complexity. Researchers then set out to acquire training data.
Bridging the gap
Samples of both pancreatic cancer and normal pancreatic tissue were imaged using a multiphoton microscope at 20× magnification (Figure 4a). Images were taken across the sample as a series of tiles 13 wide by 13 tall. Each tile had a field of view of roughly 300 × 300 µm, to cover a total area of 4 × 4 mm. Tiles were collected at five different depths, each separated by 2 µm, to accommodate variations in flatness, and the tile with the highest average brightness was used for analysis (Figure 4b). The acquired raw data was converted into three-channel images (e.g., RGB) to be compatible with the pretrained CNN input data size, wherein the red channel was assigned to represent the NADH-dominant signal, the green channel designated for the lipofuscin-dominant signal, and the blue channel for the SHG signal (Figure 4c).
Individual tiles were used for training the CNNs, and to enhance the data set, data augmentation techniques, such as horizontal and vertical flips, were employed. Then, the entire data set underwent a randomized segmentation process, allocating 70% for training, 15% for development, and the remaining 15% for testing purposes. Among the 49 samples included in the study, 21 were identified by a pathologist as normal tissue and 28 as tumor samples.
Before the initiation of training, each image in the data set was cropped to a dimension of 224 × 224 × 3 pixels, rendering them compatible with the four pretrained CNN models. The input for a CNN must fit the specific pixel dimensions for which an algorithm is designed. The architecture of the pretrained CNNs was adapted to suit the binary classification task. Specifically, the final fully connected layers of these CNNs, which were originally designed for the 1000-class classification task of the ImageNet data set, were replaced with a set of layers tailored for binary classification (e.g., cancerous or normal tissue). This modification involved the introduction of a sequence comprising fully connected layers, a Softmax layer, and classification layers, thereby reconfiguring the networks for the study’s specific purpose. Figure 4d presents a schematic representation of this adapted approach, illustrating the structural changes made to the original CNN architectures.
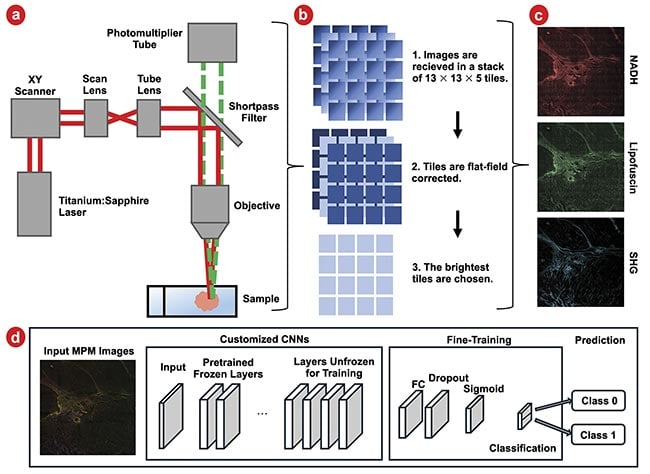
Figure 4. Samples are imaged in a multiphoton microscope (a). Images are then flat-field corrected, and the brightest layer is chosen for analysis (b). This is repeated for three imaging channels: nicotinamide adenine dinucleotide and hydrogen (NADH), lipofuscin, and second harmonic generation (SHG). Images are shown brightened and with tiles stitched together for ease of viewing (c). A schematic of customized convolutional neural networks (CNNs) with fine-tuning (d). FC: fully connected layer. Courtesy of University of Arizona.
Figure 5 shows qualitative images of diseased and healthy tissue as well as an example of a boundary between diseased and healthy tissue. Qualitatively, it is evident that tumor cells are brighter overall than their normal counterparts in the NADH and lipofuscin channels, and the tissue exhibits greater stromal striation as evidenced by the purple streaks within tumor regions. The quantitative performance of the four CNNs is depicted in Figure 6. Figure 6a encapsulates the area under the curve (AUC) for the receiver operating characteristic (ROC) curves concerning the development data set.
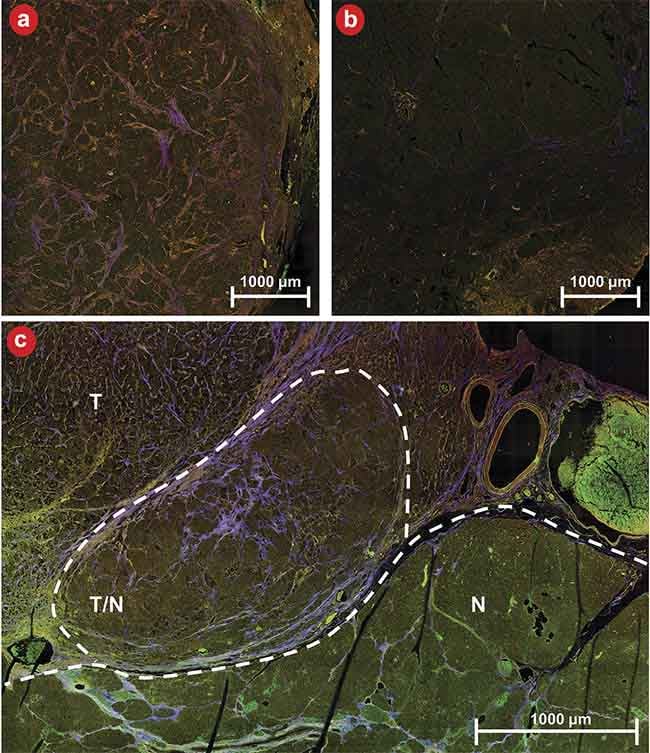
Figure 5. An example of three-channel multiphoton microscopy (MPM) images of diseased (a) and healthy (b) tissue as well as a boundary between diseased and healthy tissue (c). N: normal tissue; T: tumor tissue; T/N: region of mixed tumor and normal tissue. MPM shows strong contrast for distinguishing tissue types and margins. Courtesy of University of Arizona.
The exceptional performance of the CNNs is noteworthy, with all models boasting AUC values surpassing 0.97, with a perfect outcome being represented by an AUC of 1. This underscores the remarkable accuracy of CNNs in discerning between classes within the development set. Figure 6b represents the models’ performances on the reserved test data set. Here, AUC values remain high, all >0.95, with the maximum reaching 0.97, which are strong indicators of the models’ generalization capabilities.
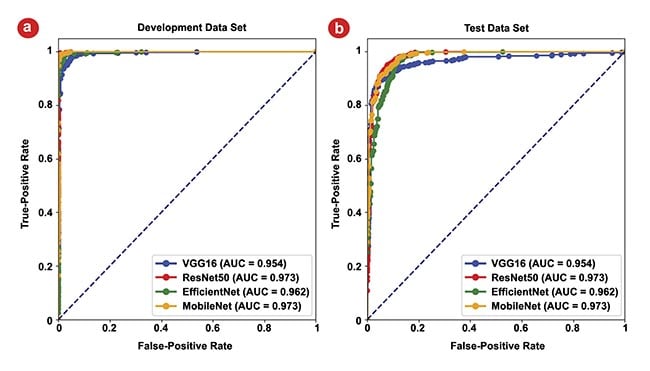
Figure 6. The area under the curve (AUC) for receiver operating characteristic (ROC) curves of the four models. The ROC curves for the development data set, where the highest AUC values for all four models approach 1 (a). The ROC curves for the test data set, all >0.95, highlighting the models’ strong generalization capabilities (b). Courtesy of University of Arizona.
Future potential
These results are extremely promising in demonstrating the potential of two-photon microscopy coupled with deep learning for providing real-time guidance for tumor margin definition during a variety of cancer surgeries. Ongoing work toward clinical translation aims to develop in vivo-capable hardware. The team is creating technology to enable intraoperative margin definition using MPM, coupled with wide-field fluorescence imaging for large area tumor localization.
In close partnership with physician collaborators, the team is working to implement capabilities in a laparoscopic system, such that these approaches could be used widely in minimally invasive surgeries. This approach involves pioneering advancements in optical system miniaturization as well as computer vision for navigation and image processing. Following the development of this device, the team will work closely with physicians and surgeons to evaluate the device through preclinical studies before transitioning to in vivo testing on patients undergoing pancreatic cancer resection.
Ultimately, the team aims to use cutting-edge innovations in optical engineering and image processing to enable better care for patients with pancreatic cancer, with the hope of broadening this approach to other cancers in the future that could benefit from improved intraoperative surgical guidance.
Meet the authors
Noelle Daigle is a Ph.D. student at the University of Arizona Wyant College of Optical Sciences. Her dissertation work investigates the use of multiphoton microscopy for surgical localization of pancreatic tumors. She received bachelor’s degrees in physics and mathematics from the University of Nevada, Reno. Her research interests are in biomedical applications of optics for cancer detection; email: [email protected].
Shuyuan Guan is a second-year Ph.D. student at the University of Arizona’s College of Optical Sciences. Her primary research area involves leveraging deep learning techniques to enhance the outcomes of biomedical imaging, with a particular emphasis on multiphoton microscopic imaging. She harbors a strong interest in blending cutting-edge AI methodologies with optical sciences to pioneer improvements in medical imaging technologies; email: [email protected].
Travis Sawyer, Ph.D., is an assistant professor of optical sciences at the University of Arizona. His laboratory’s research focuses on the development of biomedical optical technology for a variety of basic science and clinical research. He received his Bachelor of Science, Master of Science, and Ph.D. in optical sciences at the University of Arizona, as well as a Master of Philosophy in Physics from the University of Cambridge (U.K.); email: [email protected].
References
1. American Cancer Society (2023). Cancer facts & figures 2023. American Cancer Society.
2. G. Borile et al. (2021). Label-free multiphoton microscopy: much more than fancy images. Int J Mol Sci, Vol. 22,
No. 5, p. 2657.
3. A.C. Croce and G. Bottiroli. (2014). Autofluorescence spectroscopy and imaging: a tool for biomedical research and diagnosis. Eur J Histochem, Vol. 58, No. 4, pp. 320-337.
4. L. Alzubaidi et al. (2021). Review of deep learning: concepts, CNN architectures, challenges, applications, future directions. J Big Data, Vol. 8, No. 53.