Substituting a photonic tensor core for existing digital processors such as GPUs, a pair of engineers from George Washington University (GWU) has introduced a new technique for performing high-level neural network computations. In the approach, light energy replaces electricity, processing optical data feeds at a performance rate two to three orders higher than with an electrical tensor processing unit (TPU) and supporting unsupervised learning and performance in AI machines.
Neural networks commonly perform and advance machine learning, meaning the discovery has potential to develop artificial intelligence for a variety of applications.
Neural networks in machine learning are trained to classify unseen data and make unsupervised decisions based on information. Once trained on that data, a neural network can formulate an inference to identify and classify objects and patterns giving data a unique signature.
In the new system, the photonic TPU serves to improve both the speed and efficiency of existing deep learning paradigms by performing multiplications of matrices in parallel. It relies on an electro-optical interconnect, allowing an efficient reading and writing of the optical memory, and the TPU to interface with additional architectures.
Mario Miscuglio and Volker Sorger, both from the Department of Electrical and Computer Engineering at GWU, published the work in Applied Physics Review.
One distinct advantage the photonic TPU possesses involves the limitations facing digital processors in accurately performing complex operations — and the amount of power digital processors require to complete them. Neural networks unravel multiple essential layers of interconnected neurons in a process that mimics the human brain. Using a composite function that multiplies matrices and vectors together for representation, neural networks can perform parallel operations through architecture specialized in vectorized operations.
In deep learning applications, however, this can lead to overly complex networks, especially when tasks rise to a level of high intelligence or when an operation or computation requires a particularly high accuracy of prediction.
Specifically, these neural networks require considerable bandwidth and low latency. The higher the complexion and desired accuracy, the higher the amount of required power. Slow transmissions of electronic data between processor and memory detract from the applicability and desirability of digital processors.
“We found that integrated photonics platforms that integrate efficient optical memory can obtain the same operations as a tensor processing unit, but they consume a fraction of the power and have higher throughput and, when optically trained, can be used for performing inference at the speed of light,” Miscuglio said.
This combination of energy and overall efficiency along with power bodes well for the potential application of photons in the engines that perform intelligent tasks with high throughput at the edge of networks, such as 5G. Data signals at network edges may already exist in the form of photons from sources such as cameras and sensors.
“Photonic specialized processors can save a tremendous amount of energy, improve response time, and reduce data center traffic,” Miscuglio said.
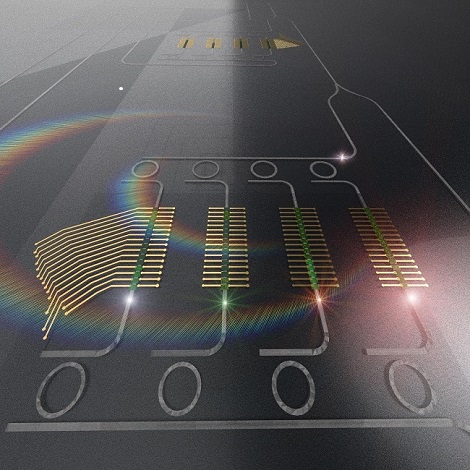
The photonic tensor core performs vector-matrix multiplications by using the efficient interaction of light at different wavelengths with multistate photonic phase change memories. Courtesy of Mario Miscuglio.
End users would benefit from increased data processing speeds since a portion of the data is preprocessed and only the leftover quantities would require the cloud or a data center.
The research was published in Applied Physics Review (www.doi.org/10.1063/5.0001942).