Machine learning makes setting up a smart camera for inspections comparable to the task of training people.
HANK HOGAN, CONTRIBUTING EDITOR
The newest industrial smart cameras feature significant advancements over their predecessors. 3D vision is becoming more common, and sophisticated onboard analytics are on the way to becoming standard. As a result, smart cameras are enabling complex assembly, surface finishing, intricate inspections, and other applications. But challenges remain, such as dealing with transparent objects, items packaged in see-through bags, and the need to make complex tasks simpler.

A robot performs pick and place. With a more capable smart camera that is equipped with a 3D sensor, the robot can perform more complex tasks. Courtesy of Zivid.
Even before anticipated advancements, today’s smart cameras come with options that are more compact and cost-effective. Tom Brennan, president of custom machine vision solutions supplier Artemis Vision, said these growing capabilities arise from advancements in hardware, and more notably from innovations in sensors.
Just a few years ago, a camera might have only had a 2D sensor along with a separate 3D sensor capable of providing x, y, and z data. This situation has changed, according to John Leonard, product marketing manager for 3D smart camera-maker Zivid.
“The amount of integration into the camera and sensors has increased considerably, I think, with 3D and 2D now present, where before it was often separate sensors. Some cameras, such as Zivid’s, also incorporate color data into their point clouds,” he said.
The extra data gathered when determining the depth of a point on an object may be needed to inspect a part and spot a defect fast enough to keep an assembly line moving. Using the 2D approach instead may require moving
a part or camera to yield an equivalent
inspection, and such movement will add to processing time, reducing throughput.
Other advancements have resulted in an increase in the number of pixels in the sensors. A few years ago, Matrox Imaging (recently acquired by Zebra Technologies) had smart cameras that topped out with 5-MP sensors. Now, the sensors range up to 16 MP. The increase in resolution makes it possible to spot smaller defects, inspect a wider field of view, or accomplish a combination of both.
Kevin Hsu, senior product manager of ADLINK Technology’s Edge Vision Business Center, said that about five years ago the company had only a 1-MP resolution in its smart cameras. Today, this parameter is as high as
8 MP, nearly an order of magnitude
increase in the resolution of the
sensor.
More pixels, more illumination
Despite having more pixels, sensors have remained the same size, avoiding
the need to reengineer the optics. Sensor vendors are making the pixels smaller, which means that each pixel is now capturing fewer photons. Although the higher-pixel-count sensors need more illumination, the increased need is slight because of enhancements to silicon.
“The vendors were clever. They improved the silicon. The pixels are more sensitive, and they have less random noise,” said Fabio Perelli, Matrox Imaging’s product manager.
Not all vendors have seen the same growth in sensor pixel count. Widely used smart camera resolutions, for instance, have changed little over the last few years, ranging from 640 × 480 pixels to as much as 5 MP.
“So far, we haven’t seen much demand for anything beyond 5 MP,” said Steve Zhu, director of sales for Asia at Teledyne’s Industrial Vision Solutions. “This could mainly be due to the limited computing power on large-resolution images in the compact smart camera platform.”

Using structured light consisting of parallel light and dark bands, a 3D smart camera performs maintenance inspection. Courtesy of Matrox Imaging.
Processing power has increased due to recent hardware innovations. Perelli said the latest Matrox Imaging smart camera, for instance, has 3× the processing power of its predecessor, without a change in footprint, the need for a fan, or the addition of any other active cooling.
In addition to general-purpose CPUs, smart cameras are now incorporating more specialized GPUs or NPUs (neural processing units) and other hardware that makes it possible to run AI-based machine learning technology on an edge IoT device such as a smart camera.
Unlike a rules-based approach that requires considerable expertise to implement, machine learning makes setting up a smart camera for an inspection application similar in concept to the task of training people. The system simply needs example images.

In a setup combining traditional machine vision and deep learning, a smart camera inspects the lips of glass bottles and spots hard-to-detect defects. Advancements make it possible for the edge IoT device to run the needed advanced analytics. Courtesy of Matrox Imaging.
“This [one] is good. This [one] is bad. This is part A. This is part B. This is a defect. This is not a defect,” said Brian Benoit, senior manager of product marketing at Cognex, to describe the system training process.
“That really lowers the barrier in terms of who can get access to this technology,” he said.
Categorizing images
The standard workflow for deep learning consists of gathering and categorizing the appropriate images, such as those of passing or failing parts. By working with these images, a machine learning system develops a model that classifies them. After validation, users can deploy this capability on a smart camera.
Machine learning is much easier to implement than a rules-based approach, which depends on engineer expertise for success. But machine learning still has requirements. In the past, a large number of training images were needed, and models were developed on a computing platform that was more powerful than a smart camera. Users also had to port the classifying algorithm from the computer to the smart camera when deploying the solution.
Today, hardware advancements have made it possible to implement the whole process on the smart camera itself. The new machine learning processing hardware is both compact and consumes little power.
“This is the perfect choice for building an AI camera for an edge application,” ADLINK’s Hsu said.
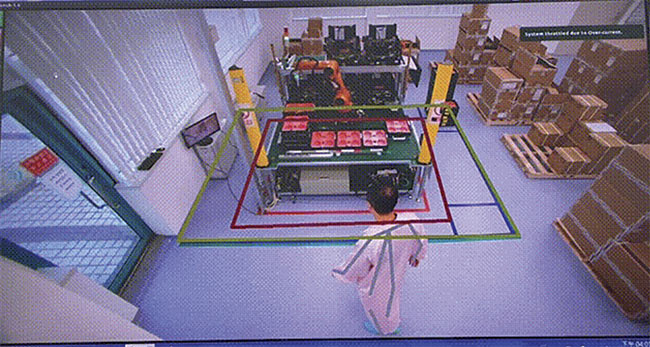
With advanced AI-powered analytics, industrial smart cameras improve safety by detecting when and where people are present near robots. Courtesy of ADLINK Technology.
Benoit said the process of training now requires far fewer images. Whereas in the past the training set may have consisted of hundreds of images, the latest hardware makes it possible for a set to be as few as five to 10 images. Thus, the training process requires less time for an expert to generate and classify images, and the machine learning workflow no longer requires having access to a server or expertise in running one.
Spotting defects
Vendors point to several examples of applications that are enabled by these hardware and software innovations. Inspecting textiles, for instance, is a difficult machine vision problem, Hsu said. Fabric often varies in color and texture, giving rise to a wide range of appearances. Spotting a defect in the midst of such variation is challenging. Yet, training and deploying a machine learning model on a smart camera can lead to classification accuracies as high as 95%, he said.
Inspecting glass bottles for defects also poses challenges. The lips of the bottles may have chips, scratches, and other damage — imperfections that are difficult to see using rules-based machine vision because of the location of the defect and the nature of the surface. In a demonstration, a machine learning-enabled smart camera was up to the task.
“The network was able to really easily detect ‘this is a perfect lip,’ or ‘this one has a chip,’ or ‘this one has a scratch,’” Matrox Imaging’s Perelli said. “You’re able to reject the ones that had a defect. With traditional machine vision this would have been very difficult because the glass is very reflective.”

A smart camera mounted on the end of a robot arm guides an automated welding process. The camera uses structured light, projecting a pattern of light and dark lines, to determine 3D information. Courtesy of ADLINK Technology.
Zhu said, “Such AI-powered smart cameras will surely fill the gap caused by the limitations of earlier smart models with rule-based traditional algorithms to identify defects with complex backgrounds.”
As a smart camera processes images, it can do more than simply categorize the information that is in them. The camera can also assign a confidence score to the image. For example, the confidence that a particular image shows a bad part could be scored at 95%. This information, in turn, can form a process quality check. Looking at trends in these numbers provides clues about how well a process is running, according to Benoit, and can uncover minor deviations before they become major problems.
The training images must be ones whose categories experts have agreed upon, he said. If no classification consensus exists, then machine learning will be unable to solve the problem, due to a lack of images that clearly fall into specific example categories.
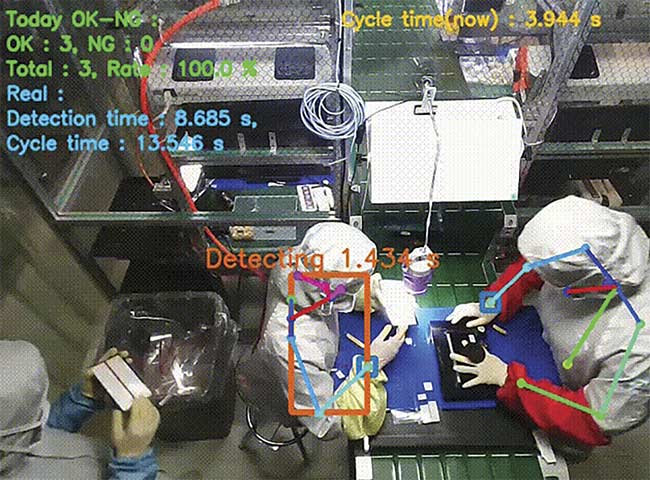
The latest smart cameras can determine compliance with standard operating procedures. Courtesy of ADLINK Technology.
Smart cameras continue to feature added capabilities, but situations still exist in which they may not be the best choice. Specific inspection tasks, for example, can require five or six cameras to capture images at various angles and thereby extract the required information. In this case, Brennan said, it may be less expensive to use dumb cameras and do the processing on a PC running a custom solution.
As smart cameras’ capabilities rise, their roles in factories will expand. The cameras may be used on robot arms, providing guidance and improving safety. Smart cameras running machine learning technology may replace fixed light curtains, allowing a more flexible safety solution.
The expanding use of AI and smart cameras, along with greater device connectivity, aid in improving diagnostics, along with process and quality control. Such outcomes, in turn, will aid smart camera vendors and their customers in using the technology.
“We will be able do more for our
customers to help [them] achieve better
uptime and better yields,” Benoit said.