Optimized lighting improves dynamic machine vision, deep learning, and IIoT applications.
Steve Kinney, Smart Vision Lights
Machine vision lighting
technology must
constantly evolve to meet increasing
demands and keep pace with advancing imaging technologies. Dynamic machine vision systems, the Industrial Internet of Things (IIoT), and deep learning are areas in which lighting innovations have enabled previously unattainable factory applications while moving machine vision beyond the plant floor.
Author Steve Kinney experiments with Ping-Pong balls and a Shop-Vac to demonstrate ultrahigh-speed image capture using an LZE300 NanoDrive light and an Allied Vision camera. Courtesy of Smart Vision Lights.
Dynamic machine vision systems are used in applications with moving or changing objects, fluctuating illumination, or changing viewpoints — for example, in active inspection applications, where robotic activity is evaluated with video in real time. Embedded computing devices, cloud computing, 3D nonvisible multisensor fusion, artificial intelligence, and event-based imaging are some of the technologies enabling dynamic machine vision. Intelligent lighting is also critical since lighting in today’s applications must be adaptable and intelligent.
Adaptable means that light properties can be changed dynamically. This includes changing intensity, moving the field of view (FOV) or focus over a coverage area to highlight a specific object, or changing angle or direction, as in the case of photometric stereo. It also involves carrying out wavelength or multispectral inspections and using polarization or other filters that may be switched in and out.
Intelligent means that the system has advanced levels of integration, control, and live data. This technology relies on optimization and plug-and-play integration with cameras and vision systems so that they all work together seamlessly. Intelligent lighting systems also provide data to Industry 4.0 systems that host or control overall processes.
Adaptive lighting can change its FOV dynamically in response to the needs of the system. Consider an FOV light with three rows of LEDs — with 10°, 30°, and 50° lenses, respectively. If channel one is on, the very narrow 10° lens is focused, resulting in the maximum projection over the narrowest area. If the 50° lens is enabled down the center channel, then the widest FOV is achieved, providing the biggest spread of light over the largest area. As one would expect, the 30° channel is somewhere in between. By mixing and matching these channels, an even, uniform coverage can be achieved, all the way from the 10° narrow to the 50° wide FOV. Unique combinations can be
used to tune and optimize lighting for applications in which fixed lighting is insufficient.
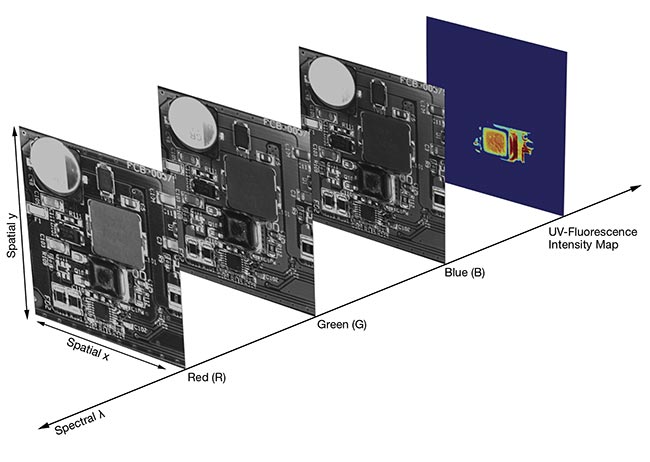
A multispectral lighting system uses four color channels (red, green, blue, and 365 nm) (top) to help produce an image with a fluorescence intensity map overlaid onto a composite color image in a real-life printed circuit board inspection application (bottom). Courtesy of Smart Vision Lights.
One example of an adaptive lighting system that may be used for color or multispectral analysis is Smart Vision Lights’ RGBW ringlight, which includes red, green, and blue channels, and a white channel for reference and to increase the color gamut. With these channels, it is possible to create
almost any visible color. Tuning enables the optimization of contrast, regardless of the color of the object or part being inspected. This delivers the
ability to get exactly the right color needed for the type of inspection being conducted.
Intelligent lights can be modified. To conduct multispectral analysis, for example, a system may need visible color lighting as well as lights in specific bands outside the visible, such as ultraviolet (UV) or near-infrared (NIR).
Some light technologies utilize only red, green, and blue, leaving many wondering about the benefit of a white channel. It is true that red, green, and blue mixed together evenly will indeed produce white, but often not a pure enough white for color or for reference. The white channel provides a reference
for color images outside of the other channels, resulting in a brighter, improved white for a color rendering index. (It also increases the color gamut — that is, the number of available colors.)
Intelligent-lighting standards
Over the last two decades, the industry has passed a number of standards focused on cameras — including GigE Vision, USB Vision, Camera Link, CoaXPress, and Camera Link HS — and the physical aspects of how data gets from one place to another. But going forward, lighting and optics support will be part of future standards. For example, GenICam, the European Machine Vision Association (EMVA) standard, addresses — in a meaningful, standardized way — plug-and-play functionality and the sockets for connecting devices so that they can communicate with one another. GenICam is now adding support for lighting and optics, enabling communication with cameras and the manipulation of certain parameters so that the host system can control a camera’s lighting and optics seamlessly.
Various types of lighting: front light (top left), diffuse light (top middle), bright field (top right), dark field (bottom left), low-angle dark field (bottom middle), and backlight (bottom right). Courtesy of Smart Vision Lights.
In 2019, the OPC Foundation’s OPC UA (Open Platform Communications Unified Architecture) specification incorporated GenICam into its communications protocol. In effect, because GenICam is present in all physical standards, it can be used in OPC UA control systems to facilitate the integration of products.
Dynamic vision in the plant
When surveying dynamic machine vision in the manufacturing plant, it’s important to recognize the components that are needed relative to the high bandwidth required. For applications in which high bandwidth is unnecessary, it is perfectly acceptable to use GigE Vision or USB Vision cameras. But when dealing with high-speed processes with movement in real time, high-speed captures at a very high frame rate are required, necessitating high bandwidth.
Cameras such as the MV4 from Photonfocus are capable of capturing many thousands of frames per second and are often coupled with frame grabbers. Frame grabbers can capture and reconstruct images using very little CPU time, freeing up the CPU to work on programming. Programmable lighting controllers further optimize the process by enabling the sequencing and control of lighting, in conjunction with a camera and frame grabber to ensure proper image capture.
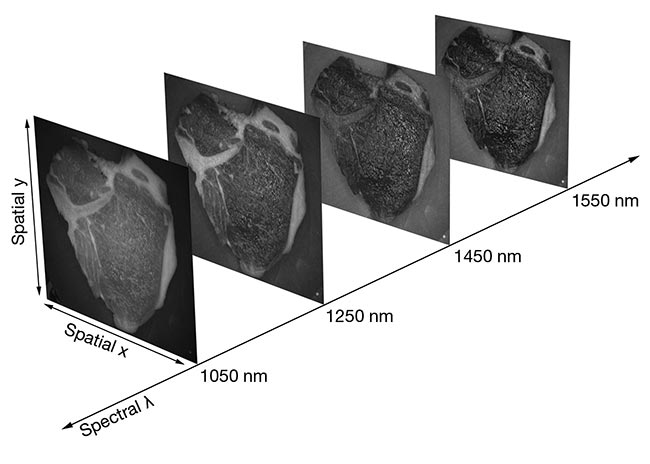
An image cube shows the results from a multispectral lighting setup comprising shortwave-IR LEDs and a grayscale InGaAs camera (top) that resolved various features of a raw pork chop, such as fat, muscle, and bone (bottom). Courtesy of Smart Vision Lights.
As plant processes increase in speed, it is important that lighting can respond, turning on and off fast enough for high-speed pulses, which are very short, to capture motion. To support
these objectives, companies are developing drivers that are capable of delivering full power to a light in 500 ns or less. This technology allows lights to be turned on and off within hundreds of nanoseconds, providing sharp, square-wave pulses down to the 1-ms range.
Dynamic adjustments
In automotive manufacturing applications, high-speed painting of auto bodies is typically accomplished using robots. Machine vision technologies “see” the paint, examine surfaces as they are being painted, and inspect auto bodies as they are exiting the paint booth. In this process, machine-to-
machine communication and control
are major emerging trends. The complex work of determining when and where spray is dispensed requires real-time feedback and vision-guided robotics.
The cameras are not only watching the spray application in real time but are also monitoring the paint application and providing data, so that software can make dynamic adjustments as the robot arm moves. Such an application requires high-speed capture and a very high frame rate to keep up with the movement of the robot. Similar complex processes can be seen in other multispectral inspection applications, such as kitting/assembly inspection, hard-to-define defect detection, and large-area inspections in textiles.
A common challenge in logistics applications is detecting and sorting packages of varying sizes, from flat packs to 1-m boxes. Portal systems are typical in automated warehouses, where Amazon, Walmart, and Target, for example, work with extensive shipping and distribution businesses such as FedEx, UPS, and the U.S. Postal Service. But variation in the size of packages on the conveyor — from 1-cubic-meter boxes to small envelopes — can be problematic, because the portal is located at a fixed distance and height from the belt. The lighting and focus that are optimized to catch the wide top of a 3- or 4-ft box as it goes by very near the camera are different than the lighting and focus settings required for the vision system to see a much smaller flat pack or envelope that is flush on the conveyor.
To address depth of focus, cameras must make adjustments. The solution
is a tunable FOV light with wireless
control. Using a box height detector/profiler coupled with liquid lens autofocus, the system knows where the surface of a box is and the box’s width as it comes down the line. This information is then used to send a control
voltage to the liquid lens focus at the right height for the surfaces being presented and to tell the light to position the FOV over the top of the box.
The hardware looks standard, but it is dynamic and being controlled by the other components within the system. It leverages the benefits of advanced LED lighting technology in its ability to turn on very fast — in less than 500 ns — while the boxes move along at a high rate. The LED technology provides up to 200,000 lux to achieve the intensity needed to freeze the motion at that rate.
Dynamic color inspection
Dynamic color inspection is important in applications where a product of a single color must be inspected for blemishes, punctures, gouges, or other defects while it is being processed and packaged. The solution involves using an RGBW light (or a multispectral light if NIR is being used) and a monochrome camera. Monochrome allows for the highest sensitivity and resolution due to the absence of color filters. Variable light allows for optimization of contrast for the various colored products or materials being presented.
For example, a food processor may be running green peppers in the morning and red peppers in the afternoon. Color tunability allows operators to change the product while using the same system and retaining the optimized light wavelength. With a monochrome camera and narrow parameters, one simple algorithm can handle all the pepper colors, giving the highest accuracy for detecting fine details.
AI, deep learning, and the training of smart cameras are other emerging trends in machine vision technology. A common misconception is that deep learning can eliminate or reduce the need for optimized lighting and high-quality images. However, several studies, including one from Arizona State University1, have indicated that this is not the case.
In general, although deep neural networks (DNNs) perform on par with (or better than) humans on good-quality images, the performance of DNNs is much lower on images with quality distortions such as blur and noise. Poor lighting configurations will result in poor feature extraction and poorly trained models. This can result in confusion about the identity of an object and what constitutes a defect. After all, AI and deep learning are akin to human learning — and they can’t compensate for poor light.
Beyond the factory floor
Dynamic machine vision applications are emerging in new areas such as augmented and virtual reality (AR/VR), digital cinema, agriculture, and drone inspections. In France, for example, the agriculture technology company Bilberry uses machine vision to identify weeds in its field-spraying applications. With multiple inspection stations assembled along a spray boom, the company can selectively apply pesticide, targeting only the weeds of concern. This enables Bilberry to use less pesticide and reduce pesticide contamination.
Machine vision is also becoming important in entertainment fields. Relighting applications are major components of complex AR/VR platforms such as Oculus and Facebook Reality Labs, as well as video conferencing tools and film production. In these applications, each light point (there can be hundreds) has a camera and a light associated with it. They light the surface of a scene from all points to gather data, and they then run massive calculations that enable the platform to place a subject in any kind of environment, even though the model was captured only once.
At the heart of dynamic machine vision is fast, accurate lighting. The technology is capable of handling high relative motion within frames that require brighter light and shorter exposure. Adaptable lighting receives and changes light according to the dynamics of the application. Finally, the networkability of smart lighting makes it ideally suited for IIoT and the standards that govern it.
Meet the author
Steve Kinney is director of training, compliance, and technical solutions at Smart Vision Lights. Prior to his current role, Kinney held positions at PULNiX, Basler, JAI, and CCS.
He is a current member of the A3 board of directors and
is a past chairman of A3’s Camera Link Committee; email: [email protected].
Reference
1. S. Dodge and L. Karam (2016). Understanding how
image quality affects deep neural networks, Arizona State University.