Thermal cameras are actively deployed on aerial and ground vehicles, in watchtowers, and at checkpoints for surveillance purposes. More recently, thermal cameras are becoming available for use as body-worn cameras.
Researchers at the U.S. Army Research Laboratory (ARL) have developed an artificial intelligence and machine learning technique to fill a specific capability gap of traditional thermal cameras: low-light and nighttime face recognition. This development could lead to enhanced real-time biometrics and postmission forensic analysis for covert nighttime operations and has direct applicability for military, law enforcement, and intelligence communities. Thermal cameras’ ability to perform automatic face recognition at nighttime, for instance, can inform a soldier that an individual is someone of interest, such as someone on a watch list.
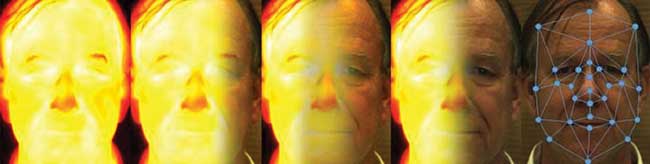
A conceptual illustration for thermal-to-visible synthesis for interoperability with existing visible-based facial recognition systems. Courtesy of ARL/Eric Proctor, William Parks, and Ben Riggan.
Using cooled polarimetric LWIR cameras developed by Polaris Sensor Technologies Inc. under an Army Small Business Technology Transfer program, research scientists Ben Riggan, Nathaniel Short, and Shuowen Hu found a solution to the nighttime facial recognition issue by enhancing both automatic and human-matching capabilities.
Riggan told Photonics Media that the technology has the ability to demonstrate current cross-spectrum face recognition capabilities using conventional thermal imagery and emerging capabilities using polarized thermal radiation.
“The polarimetric information complements conventional thermal imagery by enhancing geometric and textural details that are useful for face recognition,” Riggan said. “We demonstrated the capability for existing conventional thermal imagery acquired using the same sensor and then showed how the polarimetric state information can enhance performance.”
Conventional cameras in the field can’t capture facial imagery in low-light or nighttime conditions without active illumination, such as a flashlight or spotlight, which would give away the position of a surveillance camera. Thermal cameras work well in such situations because they capture the heat signature emitted from living skin tissue. But they are not efficient in capturing up-close facial features.


The discriminative results from the thermal-to-visible synthesis developed by ARL researchers. The bottom row demonstrates results for conventional thermal-to-visible synthesis.
The row above that shows results for polarimetric thermal-to-visible synthesis. These results are compared with corresponding ground truth visible imagery and input thermal imagery. Courtesy of ARL.
The challenge is bridging the gap between visible facial imagery, which contains a lot of detail and distinguishing features, and thermal facial imagery, which is much more obscure and degraded. Riggan and fellow ARL researchers alleviated this problem by developing advanced domain-adaptive algorithms that combine global information, such as features across the entire face, with local information to include features from discriminative fiducial regions.
They showed how the thermal-to-visible mapped representations from both global and local regions in the thermal face signature could be used together to synthesize a refined visible face image.
“When using thermal cameras to capture facial imagery, the main challenge is that the captured thermal image must be matched against a watch list or gallery that only contains conventional visible imagery from known persons of interest,” Riggan said. “Therefore, the problem becomes what is referred to as cross-spectrum, or heterogeneous, face recognition. In this case, facial probe imagery acquired in one modality is matched against a gallery database acquired using a different imaging modality.”
The approach leverages advanced domain adaptation techniques based on deep neural networks. The fundamental approach is composed of two key parts: a nonlinear regression model that maps a given thermal image into a corresponding visible latent representation and an optimization problem that casts the latent projection back into the image space.
The ARL researchers’ approach preserves identity information to enhance discriminability, ultimately bringing faces out of the dark.