In recording diverse interactions, Ozdemir set out to determine the distances between individuals – of both genders and from various age groups and cultures – whether in pairs or in larger groups, walking or standing.
Lynn M. Savage, News Editor
Whether atop microscope slides or inside shopping malls, people and objects are constantly in motion, and savvy researchers around the globe are watching closely, using digital cameras and, often, specialized analytical software to gain information on what they see.
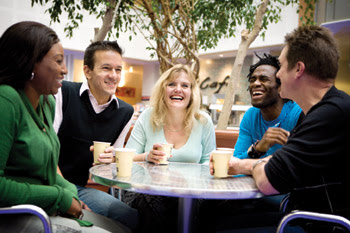
Digital photography and metrology tools are helping to measure interactions between people in venues such as shopping malls, offices and transportation centers.
One such observer is Aydin Ozdemir of Ankara University in Turkey. Visiting malls in both Ankara and in Raleigh, N.C., Ozdemir recorded people in pairs and larger groups to see how they interacted with each other within defined spaces. In the August 2008 issue of Field Methods, Ozdemir notes that architects need to know about such personal interactions to design spaces that put people at ease and that encourage them to be more cooperative, more engaged in activities and more socially involved. Putting people at ease is a factor not only in creating cubicle farms and hotels but also, when it comes to shopping malls and plazas, in garnering extra sales.
In recording diverse interactions, Ozdemir set out to determine the distances between individuals – of both genders and from various age groups and cultures – whether in pairs or in larger groups, walking or standing. To do this, he identified similarly structured open and closed-in spaces in two Turkish and two Raleigh-area malls and imaged each area for 30 minutes, taking one picture every 10 seconds. People being photographed were unaware of the camera, and they entered and exited the imaging area at will.
Once Ozdemir had finished collecting images, he downloaded them onto his computer and set about to analyze the results. Each subject in each frame was located on a floor plan of the mall, and changes in interpersonal distance were recorded automatically. More than 3000 such distances were documented at the four shopping centers.
From images recorded in the open areas of both Turkish and US malls, he found that distances between people ranged from about 24 to 120 cm. In closed areas, the range changed to 36 to 102 cm. In all, however, US mall-goers kept an extra 9 cm apart compared with their Turkish counterparts.
Ozdemir also found that male–male (69.4 cm) and female–female (68.8 cm) pairs did not interact as closely as male–female pairs, who moved in to about 64.6 cm. In addition, he found that young adults interacted with one another at shorter distances than did older adults but that, among each other, adolescents stayed most apart.
According to Ozdemir, although the results don’t explain the psychology or cultural factors behind why interpersonal distances change according to setting, knowing what those distances are in open and closed architecture could have ramifications for future building, landscape and interior design.
What are you doing?
But what, exactly, are those people doing in the mall? Or office space? Or airport? Is he reaching into his jacket for his passport, or is that a weapon? Is she gesticulating to emphasize a point, or is she about to slap a child in anger?
The best way to know in advance whether a crime or violence is about to occur is to have trained observers on the scene, but it is impractical to dot the landscape with hired watchers. Surveillance cameras can help but must be monitored and still are used mostly to catch crimes in progress – picture guards gazing attentively at banks of monitors in a high-security zone, like a casino with cameras mounted above every game table or other place where money changes hands.
Machine vision is not yet ready to help judge fast-paced crimes and misdemeanors, but the first steps in getting it ready to distinguish one motion from another are starting to take place. While completing his studies at the GIPSA lab in Grenoble, France, Emmanuel Ramasso helped develop a new algorithmic technique that allows a computer to recognize some of the actions that humans are performing on recorded video.
Ramasso became involved in the field of human behavior recognition for two reasons – because interest in surveillance technologies has increased since the terrorists’ attacks of Sept. 11, 2001, and because GIPSA has an institutional interest in indexing the content of digital videos, which nearly always involve people in motion. Ramasso and his colleagues, for example, developed their method using televised video of track and field meets, including the pole vault, long jump, triple jump and high jump events. They reported their findings in the January 2008 issue of Pattern Analysis & Applications.
When you or I watch these competitions, we quickly intuit what we see, based on previous knowledge of what jumping means and what poles are. If we haven’t seen a particular sport previously, we use contextual clues within the images, listen more carefully to the announcers to get more information, or research the topic outside of the viewing experience. A machine vision system doesn’t have these resources. Instead, it must be trained to pull information from the raw images themselves, and until now most researchers used algorithms based on probability theory – if one pixel moves from point A to point B, what is the probability that it is part of a representation of a human performing a motion?
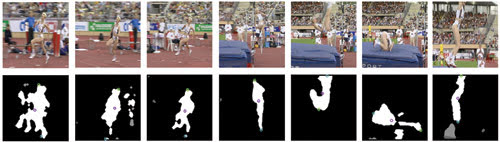
Without any prior information available to it, a machine vision system detects a pole vaulter going through a performance while ignoring background noise such as the spectators, other athletes and judges. Courtesy of Pattern Analysis & Applications.
Ramasso’s group eschewed versions of probability theory to derive a more robust way to get a computer to differentiate between a high jump and a pole vault. Instead, the team members used an analytical system called transferable belief modeling (TBM), which assigns values to pixels, then processes these values in a way that approximates how a person will intuit a possible future action rather than base every decision on the flip of a coin.
According to Ramasso, now an assistant professor at FEMTO-ST in Besançon, France, TBM enables automatic detection of human behaviors in videos, even given continuous and blurred aspects of typical motions as well as the “noise” from background motions, such as from crowds in stadiums or from other track athletes or judges.
“The transferable belief model is able to model any type of knowledge with prior [information], and this is very interesting in many applications,” he said. “Analyzing videos with TBM is promising since sometimes we have knowledge about the videos and sometimes not; mixing both is easy with TBM but more complicated with probability. TBM can process probability, but not the reverse.”
Pacing the cell
Deep inside the mall shopper, the pole vaulter and everyone else travel the cells that are the building blocks of all life. Cell biologists of all disciplines study the structures – from blood corpuscles to neurons to tumors – to elucidate what makes them work and what makes them stop working normally, leading to disease and death.
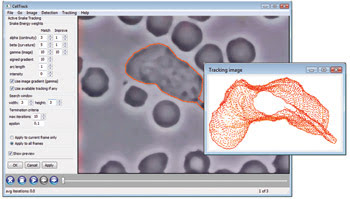
Open source software can track the natural movement of cells, providing a glimpse of the biological mechanisms leading to cancer and other diseases. Courtesy of Ahmet Sacan, Middle East Technical University, Ankara, Turkey.
Cell motility, as such movement is commonly called, is an essential part of many biological processes, according to Ahmet Sacan of Middle East Technical University back in Ankara.
“There is a growing interest in the analysis of cell motility as a diagnostic tool, especially in the areas of inflammatory disease and cancer metastasis,” he said.
While a visiting scholar at Ohio State University in Columbus, Sacan was astonished that his colleague Huseyin Coskun had to manually annotate sequential images of his subjects (amoeboids). Sacan decided to develop cell-tracking software that would help not just Coskun but other researchers as well.
Existing algorithms follow the movements of cells through a fluid by finding their outlines and identifying them in subsequent frames of a micrographic movie. Sacan says that this method leaves out important information. CellTrack, an open source program developed by Sacan, Coskun and their colleague Hakan Ferhatosmanoglu of Ohio State’s department of computer science and engineering, also tracks changes in the arrangement of a cell’s internal components and in deformations of the cell’s surface.
“Deviations from normal behavior are often characterized in terms of the cell’s velocity, trajectory and shape deformation during its movement,” Sacan said. He added that changes in these features can act as predictors of metabolic processes, such as inflammatory cell recruitment or cellular reactions to environmental cues.
As described in the July 15, 2008 issue of Bioinformatics, the software uses template matching and continuously adaptive mean shifting as its primary tracking methods. With the former algorithm, a cell in one frame is searched for in the next by identifying its outline in the first frame, sliding a copy onto the next frame and searching for the closest match. In the latter, the program finds the center of the cell in the second frame by using a back-projection of a histogram generated from the first frame. Template matching is most useful for tracking translational motion; continuously adaptive mean shifting can track rotational motion as well.
The researcher made the program open source so that cell scientists could quickly add features suited to their own work.
“There are a number of extensions that we are planning to implement,” Sacan said. “My future research in cell tracking is being shaped through collaboration with other groups to address specific research problems.”