For manufacturers specializing in short-run or seasonal products, it is often too costly to fully automate inspection processes. In such cases, AI-based visual inspection tools can help to support decisions made by human operators.
ED GOFFIN, PLEORA TECHNOLOGIES
When it comes to inspection tasks, humans do a pretty good job. We rely on our senses to detect differences, and even on taste and smell, depending on the product. If we spot a product difference or deviation, we are adaptable and can make a quick decision on our own. We are also easy to train, and we learn by example.
However, when we get tired, distracted, or bored we start making mistakes. Our eyes can also trick us. For example, try to count the white dots in the image to the right.
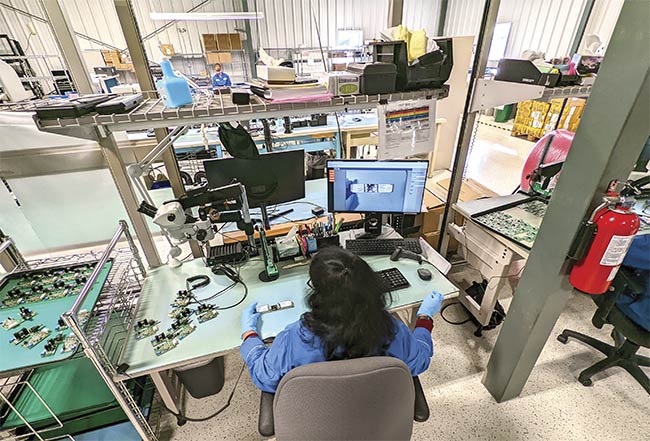
DICA Electronics uses AI-based decision support for short-run and customized solutions. Courtesy of Pleora Technologies.
Traditional vision inspection has error rates as high as 30%. Often, what an inspector flags as an error is actually a false positive because the inspector had started to doubt his or her decision-making.
The key advantage with using technology is that it can make decisions more quickly and consistently, and repeatedly, without getting tired or bored. Now, with artificial intelligence (AI), machines can begin to learn and then make decisions. Despite these machine advantages, there is still a significant need for humans in inspection processes. For manufacturers specializing in short-run, customized, seasonal, and regional products, it is often uneconomical to fully automate inspection processes. Premium and boutique brands also place a lot of emphasis on maintaining an element of human touch in their manufacturing. For these manufacturers, AI-based visual inspection tools can help by adding decision support, so that an operator always makes the right, objective choice.
New AI-based tools for visual inspection take advantage of advancements in edge processing and simplified algorithm development to help highlight product differences and deviations. For many manufacturers that are evaluating AI, traditional model training and development pose significant cost and expertise barriers. In comparison, new tools use operator input to help train the AI model. Using a basic image-compare function, the system visually highlights possible product differences for operators as they evaluate products against a saved “golden master” reference image. As an operator accepts or rejects initial possible errors, behind the scenes the AI model is being transparently trained, and it will quickly begin suggesting if it thinks a difference is an error.
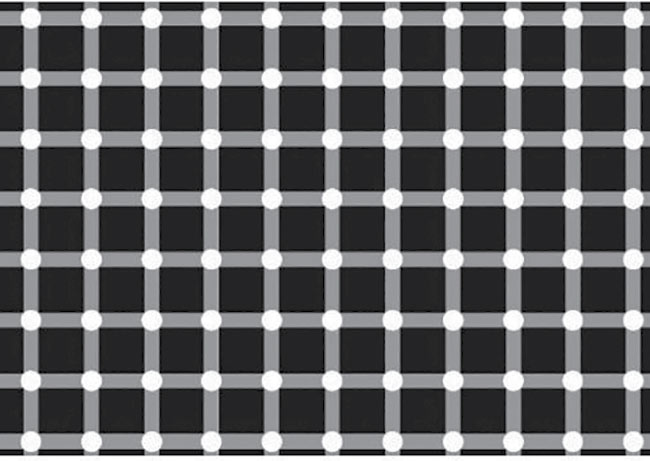
Try to count the white dots. Humans do a good job at inspection, but the eyes are easily tricked. Courtesy of Pleora Technologies.
In addition, automated and customizable reporting tools help manufacturers to gain data on visual inspection processes. Often, visual inspection poses a “data black hole” for manufacturers, which makes it difficult to ensure end-to-end quality and time-consuming to resolve issues when errors occur. For example, with these reporting tools, operators can capture images of manually inspected products alongside their notes and save the data to their manufacturing planning system.
PCB inspection
One key area where visual inspection is still critical to quality control is in electronics inspection. While mass-produced consumer goods benefit from machine vision, there is still a place for skilled human inspection of custom or short-run products for which full automation is too expensive or complex. In addition, while automated optical inspection catches most errors, it may miss issues such as component orientation, through-hole placement, labeling, and soldering defects.
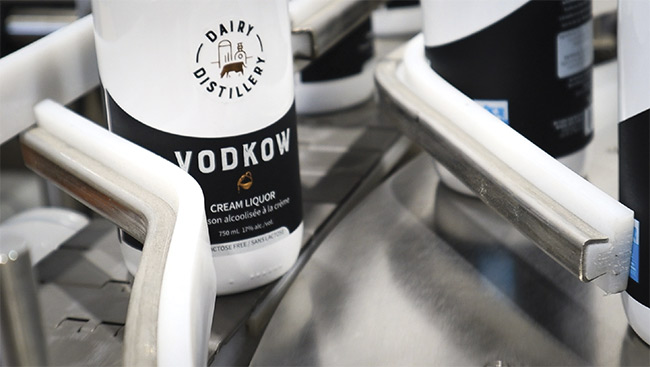
Dairy Distillery uses AI-based decision support to help protect its brand as the company competes in a global market. Courtesy of Pleora Technologies.

AI software ensures consistency in human inspection. Courtesy of Pleora Technologies.
DICA Electronics is a specialized electronics manufacturer with expertise in smaller-run, customized products for high-value, high-reliability applications. The company has adopted AI-based decision-support tools for products not well suited to automated optical inspection, or as a secondary inspection following manual processes. The intent is not to replace the human inspectors, but to help ensure they are making consistent, informed decisions even as their attention to detail begins to fade over a long day. Additionally, new products or inspectors can be more easily added to the production line, with AI ensuring the same consistent decision is made.
DICA has trained the system with a number of golden reference images for manually inspected products. As part of the training, the operator’s own early decisions on possible defects have helped train and fine-tune the AI model. As the operator and quality manager gain confidence in the accuracy of the system, they can stop AI model training. The company is then able to start using AI without requiring algorithm development expertise.
As important as error detection, the system’s integrated reporting tools provide the manufacturer with key data surrounding manual inspection processes. DICA uses the image-save function included in the reporting capabilities to capture a photo of every manually inspected printed circuit board (PCB) and its barcode, along with any operator notes. Data is then saved to the company’s manufacturing resource planning software, along with data from its automated inspection processes. If an in-field error is reported, the manufacturer can then verify whether a good part left the facility, to help speed resolution.
The ability to help pinpoint errors in manual processes — or to verify that a good product did leave the facility and a downstream issue is cause for concern — saves the manufacturer from numerous hidden costs. Without data, quality managers and staff are diverted to issue resolution processes, often involving numerous emails and phone calls with customers and other suppliers. Replacement parts may be shipped at the cost of the manufacturer, even though the root cause of the issue may not have been within its process or area of responsibility.
AI and brand management
AI and decision support can also help manufacturers to ensure consistency and reliability for in-process applications. Dairy Distillery uses AI decision-support tools for its labeling and final inspection processes to ensure consistent branding for its products.
The distillery is a small operation, producing about 1000 bottles per day while competing against global players with massive marketing budgets. A key differentiator for the distillery is its eye-catching labeling and uniquely shaped bottle, fashioned after a traditional milk bottle to reflect the company’s unique product that is manufactured from a dairy byproduct.
The bottle has three independent brand elements. The main label and cap sticker are both placed by a machine. A round, transparent emblem is then placed by a human, who must align the sticker with branding on the other two labels. The manufacturer had been using a machine for this process but was consistently seeing a 50% failure rate. While the manufacturer has automated other steps in its process, it was easier and more economical to revert back to a manual process for emblem placement.
Additionally, for a manufacturer of luxury goods, there is a certain appeal to allowing customers to see how human touch is part of the product. Part of the charm of visiting a distillery, sampling the products, and perhaps making a purchase is talking with the people who put their time, passion, and expertise into each bottle. It’s not quite the same experience watching a robot produce and package a product.
The challenge with manual labeling is that, over a long shift, the placement of the emblem could drift from bottle to bottle. During the packaging phase, an employee would notice the error and production would be halted as employees manually inspected bottles and manually removed and replaced the emblem.
The bigger threat for the business was when poorly labeled products would reach the store shelf, where they would reflect on the brand. Seeing a new product on the shelf, a consumer may question product quality based on a bottle with a misaligned label. Potentially, the retail buyer could also reject the product, resulting in return shipping costs and possible waste. Adding to the challenge, the distillery produces multiple products with different labels, and as it expands to global markets it faces different packaging requirements in different geographies. With all of the variation, it is easy for an operator to make a mistake.
To help the human operator be consistent, the distillery is using a customized visual inspection app. The operator places the bottle under the camera, and the app then identifies the brand elements on the machine-placed labels. On the display screen, the operator sees the real-time image of the bottle, along with a virtual grid that guides the placement of the emblem. The app can be easily trained for the various brand elements, depending on each product, and it is especially helpful for new operators and seasonal staff as they learn brand elements for the different product lines.
The visual inspection app ensures consistency and accuracy for the distillery’s valued brand. It saves the business time and money if labels have to be removed and reapplied. The distillery can confidently ship products, knowing they will properly represent the brand on the store shelves. The system is easy to train, and the quality manager or operator can update the app for
different products or for changing requirements by using a single good image. Over time, the AI model can also learn the brand requirements for the various packaging elements to automatically alert the operator about issues, including label defects.
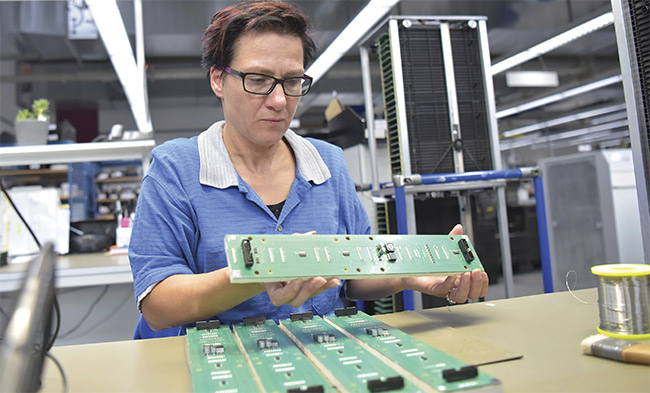
An operator at DICA Electronics inspects a printed circuit board. The operator’s early decisions on possible defects help train and fine-tune the AI model. Courtesy of Pleora Technologies.
As the distillery has tested the app, the company has provided key feedback on other areas of manual processes in which automated decision support could be beneficial to operations. An inspection pass/fail app is in development that will quickly let operators see whether labels are aligned. Down the road, the app could be trained for additional inspection of features such as fill levels, cap seal, barcode readability, and more. The manufacturer can also use the app to gather data on errors to help pinpoint process issues. These are all areas in which, if there is an issue, the product will be rejected by the store buyer, which leads to additional costs —
including replacing the product, shipping, and waste — that cut into profitability. In addition, adding decision
support helps to reduce stress for
employees who are worried about a faulty product leaving the facility.
Consistent decision-making
Programmable rules-based machine
vision excels when there is a clear difference between “good” and “bad” products. Traditional vision tools struggle, however, when decisions may
be more subjective. Products with irregular grains and patterns, for example, are difficult to assess using machine vision. Automated inspection may also have trouble distinguishing
between a scratch on a piece of hardwood floor (“bad”) and the naturally occurring grain, knots, and patterning that are desirable in high-end flooring products (“good”).
Inline inspection with AI is one way that manufacturers are adding more subjective assessment to automated inspection. This area is also one where many manufacturers rely on a human inspector to make a grading assessment. One challenge often raised by manufacturers is how to ensure consistency between operators. This is especially a concern in today’s tightening labor market, in which a manufacturer may not be able to attract and retain highly skilled inspectors, or employee turnover means the manufacturer is training new staff on a consistent basis.
The AI model can be trained to match the capabilities of a manufacturer’s best inspectors.
With an AI-based decision-support tool, the AI model can be trained to match the capabilities of a manufacturer’s best inspectors. Once the quality manager is satisfied with the AI model’s decision support, he or she can stop training the model and share the app with other inspectors. In essence, AI replicates the decision-making capabilities, experience, and expertise of the best inspector and shares it across the production facility.
AI is highly complex, with many daunting terms. This usually leads to misconceptions about costs and deployment complexity. With AI decision-support tools, training and deployment can be simplified so that any manufacturer can leverage the skills and expertise of its best inspectors across multiple production runs or facilities, or even with newly hired operators. As a result, manufacturers can start using more advanced technologies to help ensure higher quality, lower costs, and ultimately increased profitability.
Meet the author
Ed Goffin is senior marketing manager with Pleora Technologies, a supplier of AI solutions and video connectivity products for quality inspection applications. Over his 25-year career, he has been widely published and has been a featured speaker on topics ranging from packet switching and network
timing to real-time video solutions for situational awareness in medical,
factory automation, and security applications; email: [email protected].