The pharmaceutical industry relies on numerous types of inspections to ensure quality and accelerate processes in manufacturing. Though barriers to the widespread adoption of AI in this sector remain, the technology is making conventional inspections more robust and enabling new capabilities.
Visual inspections in the pharmaceutical and
medical device industries help to ensure that medications, instruments,
and packaged items arrive free of defects for end users. Whether such products are marketed for use in clinal settings or for at-home care, the processes that ensure efficiency in pharmaceutical manufacturing must perform flawless repetitions and yield high throughputs.
As a result of the heightened capabilities of AI technology, inspections have progressed from human-only pass/fail analysis — for manufactured items such as pill capsules, for example — to analyses that combine the strengths of machine vision with the strengths of machine learning.
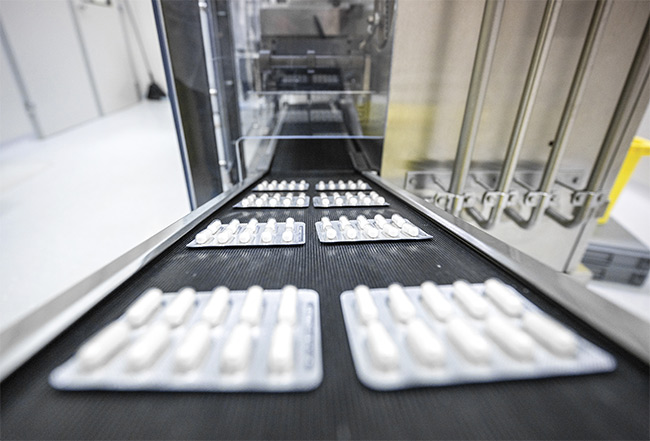
Today, algorithmic, software, and vision system advancements are enabling increasingly sophisticated AI
deployments. Where manufacturers have historically used AI to gauge differentiations in the colors of pills, systems integrators elsewhere in the pharmaceutical industry are using machine learning algorithms to gain process intelligence over the data that is output during the processes of manufacturing and health care. Hospitals and biotech companies, for example, produce
massive stores of data, and they are turning to cutting-edge software to develop deep learning (DL) models that make the information embedded in image sets usable.
The rise in AI solutions is being propelled by an increase in the population of people who require pharmaceutical care, combined with advancements in available medical technologies. As a result, the pharmacy market in the U.S. is projected to grow from $560 billion in 2021 to $861.6 billion by 2028 at a compound annual growth rate of 6.3%.

Inspections of pill capsules and blister packs containing pill capsules are applications that are well suited for AI. Courtesy of PEKAT VISION.
Year-over-year growth was already occurring in the pharmaceutical industry before 2020. The pandemic only heightened the demand on the life sciences and, in particular, on drug developers.
Enhanced capabilities
Modern AI is enabling applications up and down the value chain. Still,
AI’s role in the sector today is largely
to augment the types of visual inspections that end users have historically resolved by using rules-based machine vision or traditional machine learning methods, said CEO Ronald Müller of machine vision consulting firm Vision Markets.
An example application of vision AI involves the inspection of items that may be dangerous to consumers, such as pill capsules in the pharmaceutical
sector, he said. AI performs well in identifying anomalies and all sorts of defects.
Inspections involved in the packaging of medications are considered safety critical, and, as a result, must comply with regulations from governing or supervisory bodies. Certifying vision AI-based algorithms for safety-critical applications is a challenge. And the need for high throughputs at high speeds means using human workers to perform such vital inspections is impractical.

Deep learning and computer vision suffice for checking specifications that include shape, color, and texture. The use of more advanced AI in a semiautomated system can be deployed to gauge pill weight, which informs on contents. NOK: Not OK. Courtesy of PEKAT VISION.

The liquid-containing tubes used for COVID-19 antigen home tests. The common product component warrants visual inspection before it can be made available for retail. A vision system that performs in-line inspection determines the presence of defects. Paired with an AI component, a system provides insight into the nature of the defect. Courtesy of PEKAT VISION.
“When a manufacturer creates tens of thousands of products per minute per hour, for example, you can’t expect engineers to find critical samples and
to label those manually,” Müller said.
To certify quality inspection systems for safety, algorithms must follow transparent rules. Certifiable, rules-based
algorithms remain dominant for applications such as label inspection, even as sophisticated AI deployments increase across various sectors, he said. Deep learning is frequently used to gauge the shape, color, and surface structure of pills contained in packaging.
CEO Hongsuk Lee of Neurocle, a deep learning vision technology company, said cracks in pill capsules constitute a common problem in this area. Deep learning AI was widely
deployed for this type of inspection before Neurocle began to develop algorithms.
The company currently offers a solution for pill inspection and has developed a similar, more sophisticated AI offering for catheter inspection, Lee said. Catheter inspections require
sophisticated algorithms for product verification due to possible problems that could directly affect human survival. Such problems may include misalignment or improper positioning of the catheter tip, defects to the balloon, and problems with the catheter braid that could affect torque.
Human-assisted AI
As regulatory and governing bodies place considerable scrutiny on safety-critical inspections and manufacturing, one defining characteristic of AI in the pharmaceutical sector, according to Müller, involves overcoming the remaining barriers to unbridled adoption. End users often struggle to obtain the necessary liability insurance or governing body-issued approval to use AI for safety-critical solutions.
Müller said he is currently working with a medical device builder that is interested in using AI, but the regulatory requirements of the developer’s custom-built system will make it difficult to leave the required “good or no
good” decision without additional
input.
Jonathan Hou, president of Pleora Technologies — a supplier of AI,
embedded, and sensor networking
solutions — said he sees opportunities for AI in the pharmaceutical industry due to all the manual touchpoints that must be satisfied to complete a process. Many successful use cases are considered semiautomated, he said.
“Where we see the opportunity for AI in pharma and medical is to use it alongside machine vision and other digitization tools to help add decision support to those manual processes,” Hou said. “We want the AI to help with simplifying those tasks to ensure the operator makes the same consistent decisions.”

A misshaped pill may fail to contain an optimal dosage. Pill inspections offer a classic AI use case in the pharmaceutical and medical markets. AI mechanisms have progressed beyond detections that are based solely on shape and color, to more rigorous inspections that gauge contents and content distribution. The two types of inspections often converge. Courtesy of Neurocle.

Advanced AI is augmenting visual inspections, including those of medications. An AI-aided vision system performs a baseline inspection to identify a defect, as well as to elaborate on the nature of the defect (a bubble) and other abnormalities, such as the presence of a potential contaminant (hair). Courtesy of PEKAT VISION.
According to Tad Newman — product manager for machine vision, radio frequency identification, and precision measurement at Omron Automation — there is a fine line between using AI to enhance existing applications and using it to incite new ones.
The portion size and the distribution
of contents in a pill, for example, cannot be guaranteed without verifying the pill’s weight. Like size and shape, weight is a determining factor in a safety-critical pass or fail mechanism. Pass/fail tests are commonly used in the pharmaceutical industry to regulate inspections.
Imagers can effectively measure
surface area, but they cannot determine weight.
“That goes into the human-assisted part of the AI,” Newman said. “The human needs to say, ‘These are the features that we’re passing or failing the product based on.’ Care must be taken during system design to ensure the system is measuring or comparing the correct attributes at the right time in the process.”
Beyond inspection
The Swiss biotechnology and in vitro diagnostics company Roche uses AI in most areas of its business, the company said. AI deployments span the length of the value chain of medicines and diagnostics, from research and trials to data processing and clinical practice.
Roche’s Trial Pathfinder, co-led in partnership with Stanford University’s Institute for Human-Centered Artificial Intelligence, uses real-world data and AI to evaluate the design of clinical trials. The aim is to increase trial enrollment without compromising patient safety or the scientific rigor of the studies.
In a recent undertaking with Trial Pathfinder, Roche said studies have indicated that a vast majority of patients affected by non-small cell lung cancer failed to meet the criteria of certain clinical trials. And 86% of clinical trials failed to complete recruitment within the targeted time.
“The human needs to say, ‘These are the features that we’re passing or failing the product based on.’ Care must be taken during system
design to ensure the system is measuring or comparing the correct attributes at the right time in the process.”
— Tad Newman, product manager at Omron Automation
Roche and its partners used the Trial Pathfinder framework to emulate 10 completed clinical trials for non-small cell lung cancer and evaluated the impact of common eligibility criteria, including exclusion based on laboratory values.
Using the data-driven approach, the partners increased the total number of eligible patients in certain lung cancer trials by 107% while cutting the overall survival hazard ratio.
Roche also uses AI in drug design and development, large-volume data sorting, and image interpretation. Though individual use cases and their benefits vary by application, the company targets expediency and accuracy as common outcomes of its AI placements. To determine the viability of a prospective AI initiative, the company said it must first determine whether it has the right data to ensure that models can operate in the real world. Roche has access to a large cache of its own clinical trial data, along with that of its many partnerships.
“However, this only enables models to reflect controlled environments,” Roche said.
Other challenges include achieving “explainability” and avoiding black box models. Roche uses an expert roster of multidisciplinary data scientists, pathologists, researchers, and physicians to create, train, and validate algorithms and models, and to combat bias.
Upstream processes
More elaborate AI rollouts address
processes upstream from in-line
inspection. According to Pleora’s Hou, the origins of items such as pills and packaging must be considered, as well as any text on pills, leaflets, and labels. Leaflets that provide instructions for intended pill use, for example, must be approved by the appropriate governing body, such as the FDA. The language, placement, and shape of the text is intended to be repetitive. A single, automated process can optimize each step.
“In Europe, multiple languages must be printed on the same leaflet,” Hou said. “If you’re shipping into or out of Europe, now you have to worry about content and packaging for every single market you serve. AI and machine vision have a play there to check that all aspects of your packaging are done properly.”
Optical character recognition is adept at this type of critical text assignment.
Further upstream in in-line inspection, a robot or cobot may be unable to handle all varieties of medical kits that are distributed to hospitals. A human operator can account for nuances, such as the different items that go into one kit versus another, or the location of a label on a horizontal kit versus a square kit. When thousands of kits are being assembled, however, manual operation alone becomes less practical.
“We see potential in terms of automating or assisting a manual process using AI to check that the person is doing the right thing,” Hou said.
“AI assistance tools can guide an operator on the sequence of tasks
that they have to do so no items are missing.”
Information and operation
With humans in the picture, quality control inspections of safety-critical parts are prone to subjectivity. The job of AI is to reduce the level of subjectivity, said CEO Erin Barrett of machine vision software developer Eigen Innovations Inc.
“When you have a number of people working different shifts throughout the day, people can have different interpretations of ‘quality,’” she said. This poses a problem for quality control and assurance in a manufacturing environment.
Eigen and its partners have introduced AI solutions regarding safety-critical inspections for the automotive industry. This necessitates manufacturing applications that are common to both automotive and medical device manufacturing, such as injection molding or plastic welding. There are parallels to safety-critical inspections in the pharmaceutical industry regarding potential risks to customers if inspections fail.
Eigen also uses AI to help customers
manage massive amounts of data and to output usable information to end users. This quality also coincides with uses in the pharmaceutical industry.
Similarly, Neurocle’s core technology,
the Auto Deep Learning Algorithm, automatically optimizes parameters and architecture to create high-performance AI models, according to the company. With this technology, users who have no coding or deep learning knowledge can create AI deep learning models. The company supplies the software directly to hospitals so that they can develop the appropriate models. This enables doctor-driven medical research.
“Doctors who already have many medical images containing data can build the model by themselves,” Lee said. The solution enables the end user, in this case the medical personnel, to save considerable resources compared to those expended in a collaboration with DL engineers.
Andy Long, founder and CEO of machine vision systems integration company Cyth Systems, said using AI to contend with large volumes has emerged as a trend in pharmaceuticals and beyond.
“Because of large data sets, in the last few years I have seen AI become more acceptable than traditional
machine vision in certain areas, as the AI systems speak directly to the FDA in regard to providing large data sets that can be rerun and rechecked on a whim if a small change is made,” he said.
According to Long, AI has large image and data sets built into its DNA. Traditional machine vision lacks the concept of storing 10,000 images for any tests that may occur.
“AI has also opened the concept of cloud computing,” he said. “And this opened up the possibility of an unlimited scaling in computing power and storage.”
Furthermore, Eigen’s Barrett said, using AI for data management has collided head-on with the needs of the market.
“The bridge between operations technology (OT) and information technology (IT) is getting more connected,” she said. “How IT departments manage their software, or purchase software, is starting to become what it looks like in the operations side of the house within manufacturing.”
The results of these types of AI implementations provide an introduction to using process intelligence to predict and control in-line quality.
“You can get beyond just the value proposition of inspection,” Barrett said. “You can get to what’s causing the problem by connecting the process variation with the vision data.”
Adoption barriers and trends
AI may provide a technical advantage in the pharmaceutical industry, but it may not be a viable resource. Vision Markets’ Müller said a wealth of applications exist for which AI is not suitable because it cannot yet be certified and insured.
The time it takes to deploy an AI solution and update its deep learning models as needed is also apt to bring processes to a halt, he said. This is often a nonstarter for OEMs.
Validation is the line in the sand that determines whether and in what situations AI can be used.
“Validation means that you’re testing to make sure the system is performing up to the intended requirements, and the results are consistent and repeatable,” Hou said. “In pharma, along with many other applications, it can be challenging to train and deploy AI. There’s often not a large data set to train a model, and, especially with shorter run production, there are new scenarios for the technology to learn.”
Either because of the hurdles required to implement an AI solution, or because those who operate in the life sciences sector are unfamiliar with the capabilities of AI and machine learning, the life sciences industry is lagging behind other sectors on the AI adoption curve.
“Pharma in general isn’t an industry that wants to take a lot of risks or try things that are completely new,” Hou said.
Neurocle’s Lee said, “Compared to electronics, pharma has much more human inspection. It’s a good market for deep learning. But it’s a conservative market.”
In automotive and electronics, he said, customers are eager to jump into test trials right away. Neurocle has made inroads in the automotive space. It also operates in electronics.
“The pharma industry wants to see results first and test later,” Lee said.
The desire to automate in the pharmaceutical industry and the life sciences, however, is picking up. AI inspection company PEKAT VISION said, “These sectors are slowly beginning to wake up to the realization that AI/DL solutions bring many advantages in terms of economic benefit as well as in terms of improved consistency and quality.”
PEKAT VISION also said this could give companies operating in the sector a competitive edge.
The company offers its customers
feasibility studies as a first step to implementation. PEKAT VISION works to convey the message that, while the pharmaceutical and medical industries face stricter regulations, AI in many instances offers the best solution to satisfy these regulations.
Another catalyst for increased AI adoption, Long said, is the rise of personalized medicine. The drug tisagenlecleucel, marketed as Kymriah, was approved in 2017 as a treatment that can be customized to a patient’s unique DNA.
“This helped Big Pharma, the FDA, and biotechnology look at things
differently,” Long said. “Historically a drug was clinically trialed on a population group, and the efficacy of the treatment was determined by that group,
regardless of the intricacies of their DNA. Imagine a drug that has historically failed clinical trials, but now is targeted only at people with specific genes. The efficacy results vary tremendously now, but the data view also must change for this to be effective.”
To help personalize health care, Roche has also turned to AI. “Projects like Trial Pathfinder are central to realizing the future of personalized health care,” the company said.