From license plate reading to red-light enforcement and pedestrian safety, innovations in vision are making the roadways safer.
HANK HOGAN, CONTRIBUTING EDITOR
According to analysts at INRIX, traffic congestion cost individual drivers in the U.S. $869 in 2022,
an increase from $564 the previous year. In 2022, drivers in Germany and the U.K. lost $439 and $926, respectively, from sitting in traffic, an increase from $408 and $779 in 2021. What’s more, traffic fatalities increased by 19% between 2019 and 2022 in the U.S. and increased about half of that in Germany.
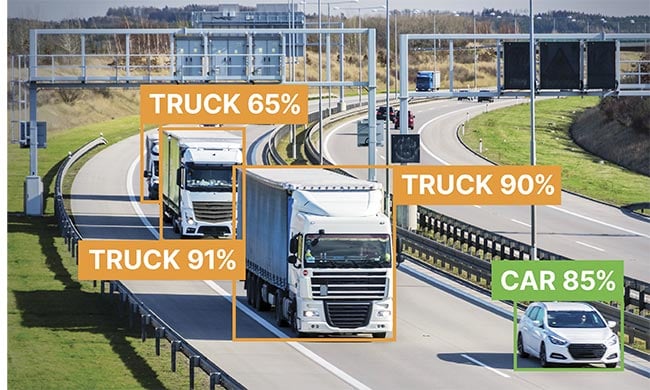
AI and machine vision
improve traffic monitoring
by enabling more accurate
vehicle identification,
benefiting applications
such as license plate
reading, toll collection,
distracted driver
detection, and more. Courtesy of Basler.
These trends of increasing congestion and fatalities spurred investment in infrastructure, with part of this money going into machine vision systems. While their costs are dropping, their performance is rising — due to better sensors, the implementation of AI, greater processing power, and other innovations. Consequently, cities and regional governments are using the technology for intelligent transportation systems (ITSs) and traffic monitoring, yielding benefits.
“You want to keep cars moving. You want to keep your carbon footprint low. You want to keep people happy going from A to Z through your town or on your highways,” said Corey Fellows, director of sales for industrial markets at Teledyne Imaging, in describing frequent optimization goals of traffic monitoring.
AI bolsters results
The use of AI and machine vision can achieve results beyond what is possible with machine vision alone. In one example, Teledyne engineers developed a license plate reading system that achieved an accuracy of 75% using only machine vision in real-world tests involving different plate designs, varying weather, and a range of lighting. By adding AI and segmenting the problem to optimize the use of traditional machine vision tools and machine learning technology, engineers developed a system that achieved 95% accuracy.
With vision systems and AI analytics, traffic planners can acquire more useful data about the current situation and in turn have more accurate insight into future conditions.
In this instance, developers trained an AI engine using a set of images with and without vehicles and plates to detect cars, trucks, and motorcycles and locate the license plates. They deployed traditional machine vision to perform the necessary optical character recognition to decipher the contents of readable license plates.
The ability to count and classify vehicles can help keep traffic moving and ensure more accurate planning to meet future transportation needs. Technology can also improve safety by, for example, ensuring that an intersection is free of pedestrians before traffic flows through.
Continued progress in traffic monitoring technology, however, may require better AI models and training. Additional advancements might be required, such as the use of a new imaging spectrum and modalities, along with other innovations.
Fellows said that the different applications that make up traffic monitoring require different imaging resolutions. Detecting and accurately reading a
license plate, for instance, requires ~100 pixels for every ~1-ft-wide plate, with many users opting for about double that resolution. This translates to a 3- to 5-MP camera. Speed and red-light enforcement applications typically use an 8- to 12-MP camera. Pedestrian safety applications in the past only needed 2 MP, but the number today
is in the 5- to 8-MP range, Fellows said.
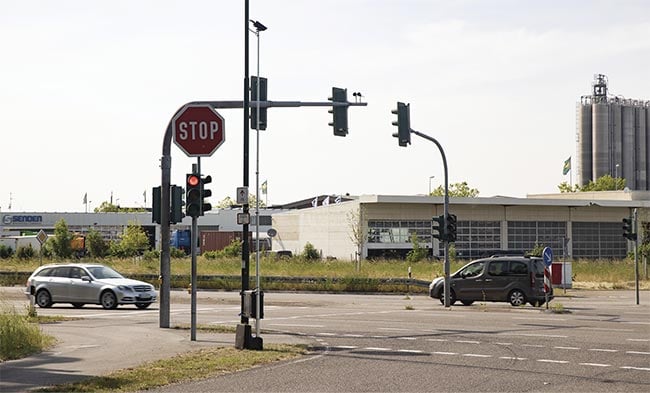
An AI-powered machine vision system mounted atop a pole classifies traffic according to vehicle type at an intersection. Courtesy of Traffic Logix.
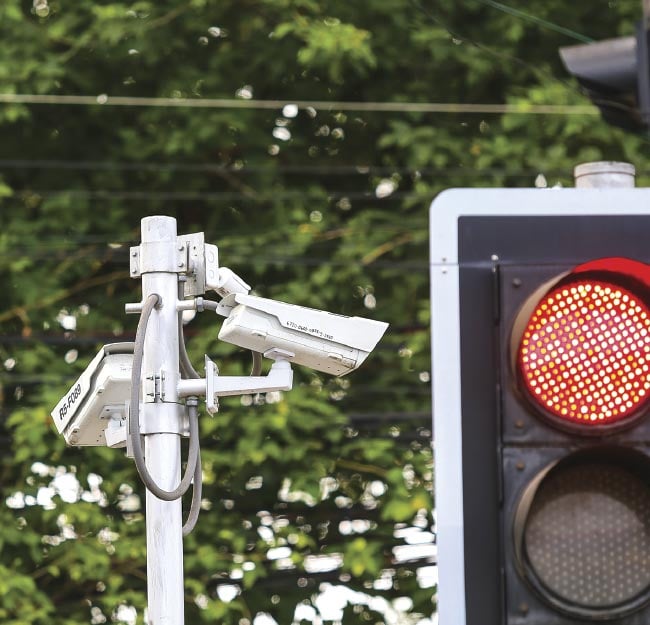
Traffic monitoring cameras operate outside
and are subject to varying weather and lighting conditions. AI software can adjust camera
parameters to improve performance in image capture and image analysis. Courtesy of Teledyne.
Pauline Lux, a Basler product group architect in software for deep learning and image processing, also recognized a trend toward higher resolution. A reason for this, she said, is a general tendency to have a vision system and associated software supporting more than one application. Thus, the system may read the license plate, a crucial step for any subsequent legal action, and may also capture the number of occupants in the car, useful for toll calculations. It can also look to see if the driver is distracted, which requires identifying the driver and determining if the driver is busy looking at a phone, for example.
“We always need the highest resolution to cover more than one application,” Lux said.
Since installing infrastructure is expensive, it makes sense to develop a higher-resolution vision system than what is currently needed. There can be drawbacks, however. Simply capturing and transmitting high-resolution images places a burden on the communications network, servers, and storage. AI can help lessen this burden by doing the processing at the edge and sending only the required images and information from the camera to the rest of the ITS.
For instance, detecting a distracted driver involves the analysis of repeated images. Some identify the car while others capture the driver looking at something other than the road. Only one image might be needed to take enforcement action, though. For example, of the license plate and a single shot of the driver looking at a phone.
The use of vision systems with analysis capabilities confers safety and other benefits that are not obvious, said Mark Gregory, senior regional manager for Traffic Logix. In addition to its own camera products, the company resells technology that classifies vehicles into 10 different categories, providing information about traffic volume, composition, and speed through an analysis of vehicle size and shape, performed using proprietary software. The output of the system, when installed properly, is almost always correct.
“In most studies I’ve seen, it’s 90% accuracy or better,” Gregory said. “It’s done instantaneously and accurately.”
The traditional method for collecting traffic data involved laying down pressurized rubber tubes over pavement, which detected passing cars as they squeezed the tubes. But this arrangement presented problems for personnel collecting the data. It also generated limited data that was subject to human error. Machine vision approaches eliminate such issues.
Vision-based systems, however,
face their own challenges. They must, for instance, operate outdoors in all weather and lighting conditions.
Tom Brennan, president of system integrator Artemis Vision, said that
vision systems exposed to the weather must be water and dust resistant while being able to perform across a temperature range as great as −40 to 80 °C. “Getting electronics to meet the temperature range is a tough challenge,” he said.
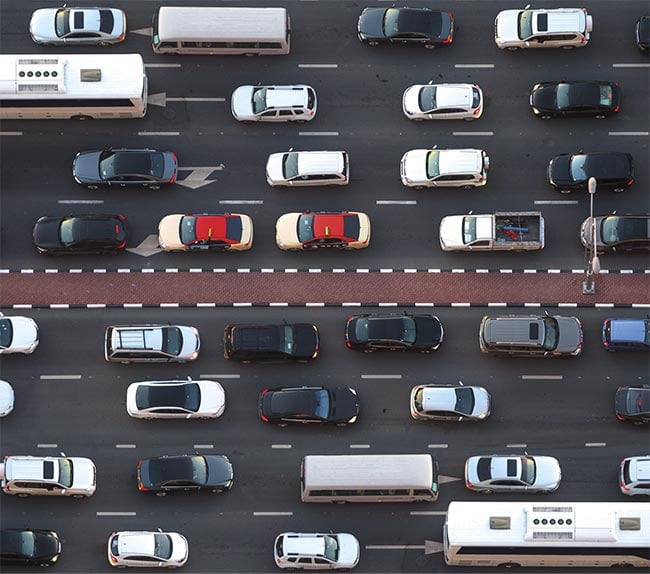
Increasing congestion is driving a need for better traffic monitoring solutions. AI and machine vision are helping to meet this challenge. Courtesy of Teledyne.
The higher end of the range might seem like something that will never occur, because it is 176 °F. But equipment might operate in this temperature, Brennan said, in the case of a dust and waterproof enclosure full of circuit boards, processing images while baking all day in the summer desert sunshine in Phoenix.
Another significant vision challenge is the lighting itself. The widely varying state of outdoor lighting affects the ability of the vision system to collect data.
System developers avoid certain
issues by incorporating filters. For
instance, Fellows said that CMOS-based sensors typically block everything past the 650-nm wavelength during daytime operation to avoid saturation from the infrared. During the nighttime, a controller automatically removes the filter, and the system uses NIR imaging, along with associated illumination, to capture data.
The material on different license plates, however, responds in varying degrees to NIR light. Thus, getting the best image may require illumination and imaging with different NIR bands.
Much more can be achieved beyond these adjustments of filters and spectral bands. Hundreds of combinations of parameters exist: Gain, optics, irises, lighting, frame rate, and more are all parameters that can affect image quality. In addition to analyzing the images, AI and machine learning allow software to automatically adjust the parameters through either settings in the camera or through external motorized optics to obtain the highest possible quality image, with these adjustments potentially performed on-the-fly in response to current conditions.
For this AI-driven enhancement, as with others that involve analysis, a key point is that the more training data that is used, and the more comprehensive the training set that the system uses to build its model, the better the results will be. “The more you train the system, the more intelligent it’s going to be,” Fellows said.
Abundance of training data
One advantage that traffic monitoring has in the rollout of AI is the myriad of training data, Lux said. This allows an easier implementation of an application that began with toll collection and evolved to flagging distracted drivers.
There is a large installed fleet of cameras and replacing them will take time and money, which would seem to indicate that the implementation of AI in traffic monitoring may be slow. According to Lux, though, it may not be necessary to completely tear out or totally redesign old hardware. With sufficient room for the software and processing power in the system, solution providers can add AI. If this is not possible, an AI accelerator board can be used to replace or supplement the existing vision system.
“AI is enabling so many more applications that we were not thinking about in past years,” Lux said.
She said that Basler supplies the software and hardware infrastructure for a solution. The traffic monitoring know-how, on the other hand, comes from the end users.
Looking forward, an area for further innovation includes expanding the spectrum that is used or combining vision with other data. At present, traffic monitoring uses color cameras, with the use of the infrared up to ~1000 nm. Being able to use the infrared region further could provide additional information and enable more accurate imaging in a wider variety of lighting and weather conditions.
Another area of expansion could be to bring data in from other sensors, such as those that detect traffic through pressure or weight changes. Such data could be important for monitoring commercial traffic, such as large trucks hauling freight.
This setup will likely result in an increasing need for processing in the vision system itself. Along with the advent of AI, this is part of an ongoing trend toward increasing computing on the edge of a network. Using edge processing in traffic monitoring enables the use of more data without overwhelming network or storage capacity. “Processing close to the edge can do some of the heavy lifting,” Lux said.
Finally, the information provided by AI and vision systems could solve a fundamental challenge in traffic monitoring. Typically, transportation planners use a heavy dose of fudge factors in their work. The data they have captured in the past has offered an incomplete picture, and so it is important to allow for the unknown when planning five, 10, or 30 years in the future. With vision systems and AI analytics, traffic planners can acquire more useful data about the current situation and in turn have more accurate insight into future conditions.
However, the one constant need across all the different monitoring applications prevails — one that the use of AI can help meet. As Gregory said, “You want a tool that’s going to give you real answers.”