Photonics HandbookBioScan
Machine Learning Enables Label-Free Prediction of 3D Images
Scientists at the Allen Institute have used machine learning to develop a label-free tool for predicting 3D fluorescence directly from transmitted-light images. Instead of fluorescence microscopy, the new method uses only black-and-white images generated by a bright-field microscope.
To the human eye, cells viewed in a bright-field microscope are sacs rendered in shades of gray. A trained scientist can find the edges of a cell and the nucleus, but not much else. Using a convolutional neural network, the team trained computers to recognize the details in the bright-field images. The researchers tested 12 different cellular structures and were able to show that their technique could be used to generate multistructured, integrated images. The model generated by the machine learning algorithm predicted images that matched the fluorescently labeled images for most of the structures.
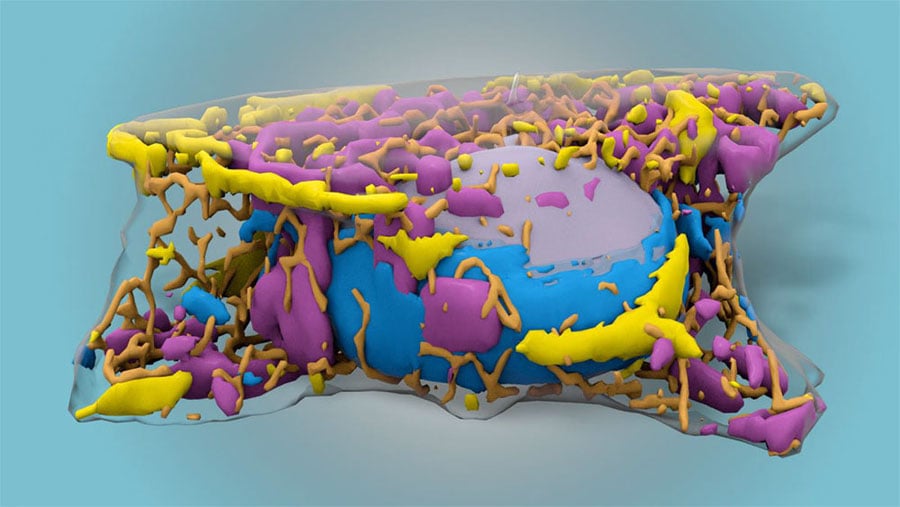
A machine learning algorithm developed by Allen Institute researchers used what it learned from cells with fluorescent labels to find cellular structures in cells without fluorescent labels. The machine-learning method uses black-and-white images generated by bright-field microscopy. Courtesy of Allen Institute.
The new label-free method can also predict immunofluorescence (IF) from electron micrograph (EM) inputs, extending the potential applications, said the team.
“Going in, we had this idea that if our own eyes aren’t able to see a certain structure, then the machine wouldn’t be able to learn it," said researcher Molly Maleckar. “Machines can see things we can’t. They can learn things we can’t. And they can do it much faster.”
The prediction tool could help scientists understand what goes wrong in cells during disease. Cancer researchers could potentially apply the technique to archived tumor biopsy samples to better understand how cellular structures change as cancers progress or respond to treatment. The algorithm could also aid regeneration medicine by uncovering how cells change in real time as scientists attempt to grow organs or other new body structures in the lab.
The prediction toolset is freely available and could lower research costs if used in place of fluorescence microscopy. Fluorescent tags are also subject to fading, and the light itself can damage living cells, limiting the usefulness of fluorescence labeling in the study of live cells and their dynamics. The machine learning approach would allow scientists to track precise changes in cells over long periods of time, potentially shedding light on early development or progression of a disease.
“This technique has huge potential ramifications for these and related fields,” researcher Rick Horwitz said. “You can watch processes live as they are taking place.… This method allows us, in the most noninvasive way that we have so far, to obtain information about human cells that we were previously unable to get.”
The research was published in Nature Methods (https://doi.org/10.1038/s41592-018-0111-2).
See how Allen Institute researchers used machine learning to create a new method for predicting 3D fluorescence microscopy images from transmitted light microscopy images. Courtesy of Allen Institute.
Published: September 2018