As labor concerns grow, food and beverage producers are turning to robotic systems. Artificial intelligence enables a range of capabilities, especially for variable items such as proteins and produce.
AUSTIN HARVEY, OXIPITAL AI
For decades, machine vision has helped robotic systems surpass limited, rote applications, enabling a wide range of pick-and-place applications across industries. One of these industries is the food and beverage industry, but the natural variability of products in this sector — especially proteins, fruits, and vegetables — presents challenges for this technology. Traditional vision-guided robotic systems perform well in food and beverage manufacturing and packaging under controlled conditions, but they struggle with irregularities in product shape, size, texture, appearance, and presentation to the operator.
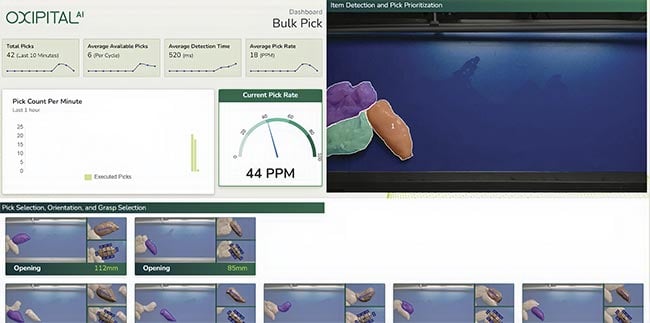
By augmenting a vision-guided robot system with AI, systems can inspect, singulate, sort, and package ears of corn. Courtesy of Oxipital AI.
It is a market worth addressing, since the need for increased efficiency in food processing places high demands on robotic systems as food processors aim to reduce waste and energy consumption. The food robotics market is expected to expand at a compound annual growth rate of 12% between 2023 and 2032, reaching $10.5 billion, according to Global Market Insights.
The introduction of artificial intelligence (AI) into robotic systems has transformed food and beverage processing. AI is providing layers of flexibility, enabling robotic solutions to adapt in real time, making them more efficient, accurate, and reliable in handling the high degree of variability inherent in food production. AI-powered vision-guided robots are revolutionizing the food and beverage industry by addressing evolving needs and demands. Whether dealing with singulated or bulk products, AI-based robots can fit into existing workflows and handle the variability challenges encountered by businesses.
AI and vision-guided robotics
Machine vision systems have traditionally allowed robots to detect objects, understand their position and orientation, and perform specific tasks, such as picking, placing, and sorting. However, traditional vision systems often rely on rigid programming and predefined algorithms that work best in predictable environments, such as automotive manufacturing, where parts are uniform, standardized, and rigid, making them easier to handle. In food and beverage manufacturing, every chicken breast coming down the line differs from the next — not the kind of rigidity that lends itself to uniform handling.
Through deep learning and neural networks, AI offers a significant improvement in how vision-guided robots perceive and interact with irregular objects. AI enables robots to learn and adapt from data, meaning they can recognize and handle products that vary significantly in size, shape, and appearance without needing manual reprogramming.
Key AI capabilities in vision-guided robotics include:
• Object recognition: AI-powered vision systems can identify different food items even with varying appearances. For example, a robot sorting tomatoes can distinguish between a ripe and an unripe tomato based on color and texture, even if the size varies, which delivers higher profitability to manufacturers and more consistent product quality to consumers.
• Flexibility in handling: Traditional robotic systems struggle with deformable products, such as a soft loaf of bread or a piece of poultry. AI helps robots make real-time adjustments to their grip, allowing them to handle delicate or irregular items without damaging them.
AI-enhanced vision systems excel in dealing with the variability found particularly in organic products, such as produce and protein. When handling fresh produce, AI can recognize deformities, irregular shapes, and varying sizes. This ability to adapt dynamically ensures that robots can still perform essential tasks — such as sorting, cutting, and packaging — even when the product does not fit a predefined model.

AI-enabled software can help singulate
individual items, such as pieces of poultry,
from bulk. Courtesy of Oxipital AI.
In packaged food and consumer packaged goods, AI is improving flexibility on production lines. Companies in these industries often must switch between different produce shapes, sizes, and materials for various product lines. AI-enabled vision systems allow robotic arms to adapt to these changes without the need for reprogramming. The system can automatically detect different product types and adjust its movement accordingly, reducing downtime and improving overall throughput.
AI in product inspection
Handling raw meat in food processing presents several challenges. Pieces of chicken, for example, can vary significantly in size and shape, making it difficult for a robot to pick and place them consistently. With AI-powered vision systems, robots can analyze each piece individually, adjusting their movements accordingly.
But AI can be put to work in food and beverage applications beyond only helping to sort and pack items effectively based on size and orientation. It can also greatly enhance inspection capabilities, such as evaluating fat content in chicken breasts or detecting spoilage or allergens that might not be visible to human inspectors.
While traditional quality inspection has relied on rigid algorithms designed to find specific defects or flaws, AI provides the ability to find anomalies despite inconsistencies in product makeup or orientation. AI adds a new layer of intelligence to machine vision by enabling robots to learn and adapt to scenarios without human intervention. Unlike traditional vision systems, AI-enabled solutions use machine learning, deep learning, and other techniques to recognize patterns, classify objects, and make decisions based on real-time data.
In the food and beverage sector, AI-driven vision systems can analyze visual information more holistically.
Instead of relying on fixed parameters, AI can learn from vast data sets of images to distinguish between normal and abnormal variations in food products.
Real-world inspection examples
Manually inspecting produce has always been a difficult task, and despite spending hours to identify and pick acceptable products, human workers often make errors. In a processing plant, the variance in the size, color, and texture of corn, for example, makes traditional sorting methods inefficient. AI-enabled vision-guided robots, however, can easily singulate, sort, inspect, and package ears of corn. Using
machine learning algorithms, a system can recognize the size, shape, and orientation of the corn in bulk, with the ability to differentiate between good and bad pieces (opening image).
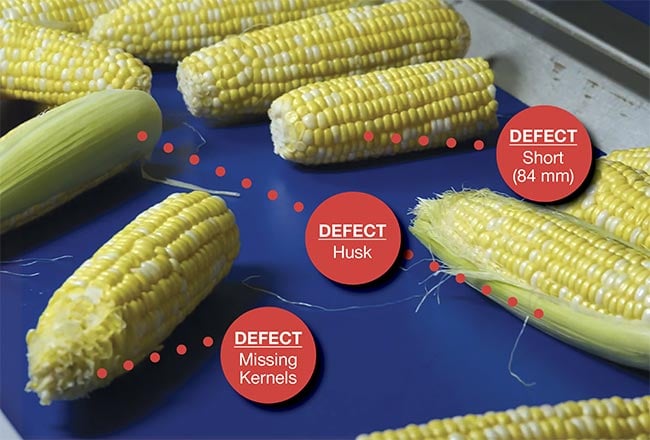
While providing visual guidance for the robot to make a pick, an AI-enabled system can also identify defects. Courtesy of Oxipital AI.
This automation not only increases the throughput of the sorting line but also improves the quality control of packaged products. By identifying imperfections, AI-powered robots ensure that only high-quality produce is packed for sale, reducing the number of defective products shipped to consumers. In addition, inspection data acquired by the system delivers quality data to manufacturers for inbound crops, which allows them to have stronger negotiating positions with suppliers.
A tomato-sorting robot powered by AI can differentiate between ripe, overripe, and underripe tomatoes by analyzing subtle changes in color and texture. This allows the system to adjust on the fly, ensuring that only tomatoes of the desired ripeness are packaged for sale while others are directed to alternative processing streams.
Benefits of AI
AI adds significant value to vision-guided robots in food and beverage manufacturing, providing several key benefits:
• Enhanced accuracy and speed: AI-enabled robots can perform tasks more accurately, ensuring that products are placed or sorted correctly. This accuracy allows systems to operate at higher speeds without compromising quality, ultimately increasing productivity.
• Increased flexibility: AI allows robots to handle a wide range of products, from uniform packaged goods to irregular organic materials. This flexibility is essential as food and beverage companies deal with seasonal changes, varying product sizes, and inconsistent supply chains.
• Reduced downtime: AI systems can adapt to changes in real time, reducing the need for human intervention. Traditional robotic systems may require downtime for reprogramming or calibration when presented with new products. AI minimizes this need, allowing production lines to operate more continuously and efficiently.
• Waste reduction: With the ability to handle delicate products and make real-time adjustments, AI-driven robots reduce product damage
and waste. This is especially important in the food industry, where even slight damage can render a product unsellable.
• Labor efficiency: As AI enhances robotic capabilities, manufacturers can reduce their reliance on manual labor for repetitive and physically demanding tasks. This improves overall efficiency, allows human workers to focus on more strategic or value-added tasks, and helps address labor shortages in the food and beverage industry.
Automation shapes the future
In the dynamic and highly variable world of food and beverage manufacturing, traditional vision-guided robots are no longer sufficient for the challenges posed by organic products, product irregularities, and increasing consumer demand for customization. AI adds new layers of flexibility, enabling robots to adapt to these variables in real time.
With AI-enabled vision systems, food and beverage manufacturers can boost efficiency, reduce waste, improve product quality, and meet evolving market demands. As these systems become more accessible and advanced, companies that adopt AI-driven robotics will gain a competitive edge in the fast-paced food and beverage industry.
As AI continues to advance, the combination of machine vision and robotic systems will only grow more sophisticated. Soon, AI-powered robots will be able to not only recognize and handle complex, variable products but also predictively adjust processes to optimize production lines. Predictive maintenance, self-learning systems, and advanced robotics will become integral parts of food and beverage manufacturing, allowing companies
to meet the growing demands of
consumers.
Additionally, AI will enable a higher level of customization. Robots will be programmed to handle specific tasks for niche products or production needs without the long setup times associated with traditional systems. As consumer preferences shift toward organic and specialty foods, manufacturers will need systems that can quickly adapt
to new product types, and AI-guided robots are well positioned to meet these demands.
The integration of AI with robotics and machine vision is not just a technological upgrade — it is a fundamental shift in how food production processes are managed, offering a smarter, more adaptive solution for the challenges of today and tomorrow.
Meet the author
Austin Harvey, vice president of product and marketing at Oxipital AI, is a seasoned product and marketing leader with more than a decade of experience in the robotics industry, specializing in bringing cutting-edge technologies to consumer and industrial markets. Under his leadership, his teams have delivered tens of millions of robots to consumer homes around the world. His most recent work at Oxipital AI has helped manufacturers deliver billions of products to their customers more efficiently while enhancing quality, improving yield, and increasing customer satisfaction; email: [email protected].